Unsupervised image classification method based on automatic encoder
An autoencoder and autoencoder technology, which is applied in neural learning methods, instruments, biological neural network models, etc., can solve problems such as cumbersome process, low precision, and high cost of manual labeling, and achieve avoidance of manual labeling, simple architecture, The effect of reducing labor costs and labor intensity
- Summary
- Abstract
- Description
- Claims
- Application Information
AI Technical Summary
Problems solved by technology
Method used
Image
Examples
Embodiment 1
[0041] The present invention takes the mnsit data set as an example. The mnsit contains 60,000 training images and 10,000 test images; the size of each image is 28*28, and there are 10 categories in total, such as figure 2 shown.
[0042] First, construct an autoencoder model as described in step S1, the compressed feature vector in the autoencoder model is set to N=3, then, take out the image in the training set, copy the image twice, and do different random transformation, such as image 3 shown.
[0043] in image 3 where a represents random translation, and b represents random rotation. Through the loss designed in step 2, the transformed image is input into the self-encoder, the loss is calculated, and the gradient is calculated through backpropagation to update the weight of the self-encoder. This loops until the autoencoder converges. Then, use the encoder in the autoencoder model to encode all the images in the test set into 3-dimensional feature vectors. The en...
PUM
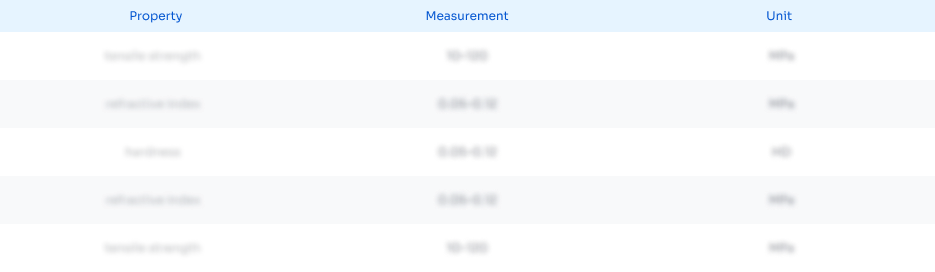
Abstract
Description
Claims
Application Information
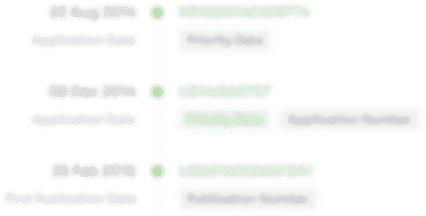
- R&D Engineer
- R&D Manager
- IP Professional
- Industry Leading Data Capabilities
- Powerful AI technology
- Patent DNA Extraction
Browse by: Latest US Patents, China's latest patents, Technical Efficacy Thesaurus, Application Domain, Technology Topic, Popular Technical Reports.
© 2024 PatSnap. All rights reserved.Legal|Privacy policy|Modern Slavery Act Transparency Statement|Sitemap|About US| Contact US: help@patsnap.com