PCB defect image recognition method based on multi-model fusion convolutional neural network
A convolutional neural network and image recognition technology, applied in the field of PCB defect image recognition based on multi-model fusion convolutional neural network, can solve the problems of high labor intensity and achieve the effect of good classification and recognition performance
- Summary
- Abstract
- Description
- Claims
- Application Information
AI Technical Summary
Problems solved by technology
Method used
Image
Examples
Embodiment 1
[0039] See body 1~ Figure 6 , a PCB defect image recognition method based on multi-model fusion convolutional neural network, the operation steps are as follows:
[0040] (1) Establish an image data set: obtain multiple types of PCB defect images, establish a PCB image data set, and classify according to each defect type;
[0041] (2) ResNet50 model improvement;
[0042] Improve the shortcomings of the existing deep convolutional neural network model, and establish a convolutional neural network framework suitable for PCB defect image recognition;
[0043] (3) Feature Fusion:
[0044] In order to further improve the effect of PCB defect classification and recognition, a PCB defect recognition method based on multi-model fusion is proposed; image features extracted based on multiple models are fused, and the output network structure of the fused features is improved;
[0045] (4) Model training to realize PCB defect identification:
[0046] The PCB picture set is divided int...
Embodiment 2
[0049] This embodiment is basically the same as Embodiment 1, and the special features are as follows:
[0050] The step (2) improves the shortcomings of the existing deep convolutional neural network model, and establishes a convolutional neural network framework suitable for PCB defect image recognition. The ResNet50 network structure consists of four large BottleNecks. Each block is composed of 3, 4, 6, and 3 small residual blocks. Each small residual block is composed of 1×1, 3× 3. Three 1×1 convolutional layers are connected in series; in addition, the front end and the last end of the network are respectively composed of a 7×7 convolutional layer, a maxpool layer and an average pooling layer. The optimized and improved ResNet50 model introduces a new type of CNN module called Res2Net, which replaces the residual blocks containing 1×1, 3×3, and 1×1 convolutional layers in the original ResNet50 with more hierarchical residual blocks. Differentiate the connection structure...
Embodiment 3
[0054] see figure 1 , a PCB defect image recognition method based on multi-model fusion convolutional neural network, which includes the following steps:
[0055] Obtain multiple types of PCB defect images and non-defect images, use various data enhancement methods to expand the image set and establish a PCB image data set, and classify it according to each defect type. The present invention selects two kinds of defects, residue and foreign matter, and after data enhancement, there are 3900 pieces of each kind of defect in the training set, and 7800 pieces of non-defective PCB images. The test set has a total of 5200 pictures, including 1300 pictures of residue and foreign matter defects, and 2600 pictures without defects. figure 2 There are two kinds of defect pictures of residue and foreign matter and no defect pictures, which are sorted out by experienced staff.
[0056] To improve the shortcomings of the existing deep convolutional neural network model, a ResNet50 convo...
PUM
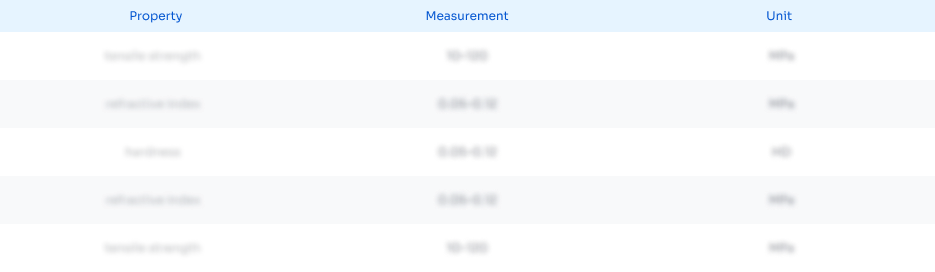
Abstract
Description
Claims
Application Information
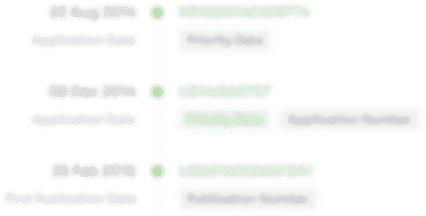
- R&D
- Intellectual Property
- Life Sciences
- Materials
- Tech Scout
- Unparalleled Data Quality
- Higher Quality Content
- 60% Fewer Hallucinations
Browse by: Latest US Patents, China's latest patents, Technical Efficacy Thesaurus, Application Domain, Technology Topic, Popular Technical Reports.
© 2025 PatSnap. All rights reserved.Legal|Privacy policy|Modern Slavery Act Transparency Statement|Sitemap|About US| Contact US: help@patsnap.com