Model privacy protection method and system for deep reinforcement learning
A reinforcement learning and privacy protection technology, applied in the field of model privacy protection based on imitation learning, which can solve problems such as attacks, data leakage security, threats, etc.
- Summary
- Abstract
- Description
- Claims
- Application Information
AI Technical Summary
Problems solved by technology
Method used
Image
Examples
Embodiment Construction
[0033] The specific implementation manners of the present invention will be further described in detail below in conjunction with the accompanying drawings.
[0034] refer to Figure 1 ~ Figure 3 , a model privacy protection method for deep reinforcement learning, the steps are as follows:
[0035] 1) Pre-train the target agent to get the target strategy π t : The deep deterministic decision gradient algorithm (DDPG) trains the car Car, and the goal of Car is to reach the destination as quickly as possible and safely. The core of the DDPG algorithm is based on the extension of the Actor-Critic method, DQN algorithm and deterministic policy gradient (DPG). The deterministic policy μ is used to select the action a t =μ(s|θ μ ), θ μ is the policy network μ(s|θ μ ) parameters, with μ(s) acting as Actor, θ Q is the value Q network Q(s,a,θ Q ) parameters, use the Q(s,a) function to act as Critic. To improve training stability, a target network is introduced for both the policy...
PUM
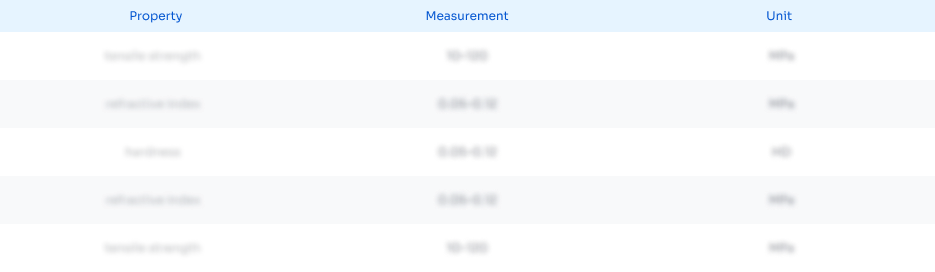
Abstract
Description
Claims
Application Information
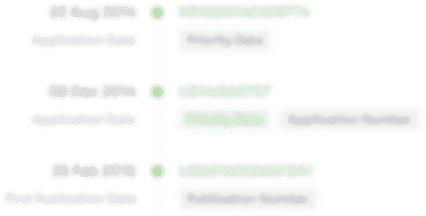
- R&D Engineer
- R&D Manager
- IP Professional
- Industry Leading Data Capabilities
- Powerful AI technology
- Patent DNA Extraction
Browse by: Latest US Patents, China's latest patents, Technical Efficacy Thesaurus, Application Domain, Technology Topic.
© 2024 PatSnap. All rights reserved.Legal|Privacy policy|Modern Slavery Act Transparency Statement|Sitemap