Reinforcement learning-based micro-grid optimal scheduling method
An optimization scheduling and reinforcement learning technology, applied in design optimization/simulation, photovoltaic power generation, electrical components, etc., can solve problems such as large impact on results, inability to guarantee optimization performance, accumulation of experience and knowledge, and achieve strong self-learning and memory. ability, improve the speed of optimization, and improve the effect of learning efficiency
- Summary
- Abstract
- Description
- Claims
- Application Information
AI Technical Summary
Problems solved by technology
Method used
Image
Examples
Embodiment 1
[0054] The embodiment of the present invention further considers the basic conditions and decision-making factors of the optimal scheduling of the microgrid. The essence of optimal dispatching of microgrids is to use the optimal energy dispatching strategy to satisfy distributed power generation requirements such as wind and wind, through the joint decision-making of controllable components in microgrids and large power grids under the basic conditions of known distributed power generation and load requirements such as wind and wind. The difference in energy between the output of a source and the demand of a load. The output and load demand of distributed power sources such as wind and solar are affected by climate and user behavior habits respectively, and climate change and user behavior habits are related to geographical location. Although the two have strong uncertainties, the same region and adjacent regions Therefore, the output and load demand of distributed power sourc...
Embodiment 2
[0125] In order to verify the technical effect of the method of the present invention, this embodiment conducts further verification through simulation experiments.
[0126] This experiment selects the prediction data of radiation intensity and user consumption from the GitHub project, and the wind speed prediction data of the wind energy database project.
PUM
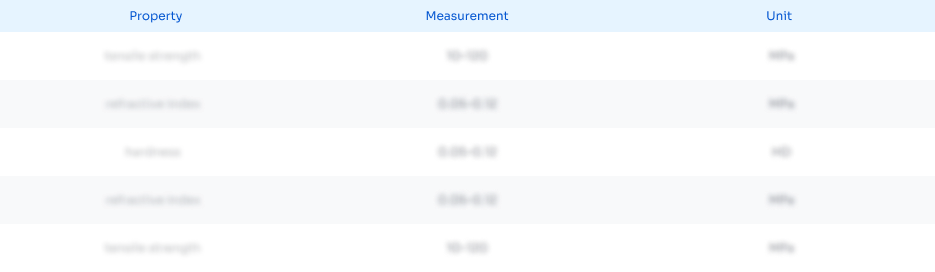
Abstract
Description
Claims
Application Information
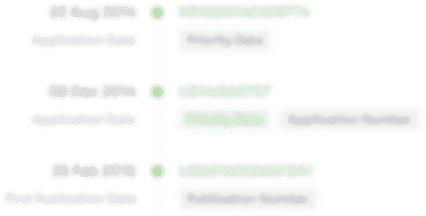
- R&D Engineer
- R&D Manager
- IP Professional
- Industry Leading Data Capabilities
- Powerful AI technology
- Patent DNA Extraction
Browse by: Latest US Patents, China's latest patents, Technical Efficacy Thesaurus, Application Domain, Technology Topic.
© 2024 PatSnap. All rights reserved.Legal|Privacy policy|Modern Slavery Act Transparency Statement|Sitemap