Encrypted malicious traffic detection method, detection system and computer equipment
A detection method and technology for malicious traffic, applied in transmission systems, neural learning methods, biological neural network models, etc., can solve the problems of unawareness of the difference between upstream and downstream traffic, difficulty, poor traffic monitoring effect, etc. The effect of good generalization performance and good classification performance
- Summary
- Abstract
- Description
- Claims
- Application Information
AI Technical Summary
Problems solved by technology
Method used
Image
Examples
Embodiment 2
[0152] 1. Public dataset CICIDS2017
[0153] The CICIDS2017 dataset captures five days of traffic in real environments, including benign normal traffic and common attack traffic, providing real traffic data (pcap files), and network traffic analysis results (csv files) based on CICFlowMeter, including time stamp, source and destination IP, source and destination port, protocol and attack type, etc. The present invention first utilizes the SplitCap traffic segmentation tool to segment the entire pcap according to the five-tuple information, and then traverses the segmented files according to the time stamp in the CICFlowMeter analysis result to segment the granularity of the session. Attack subcategories The present invention merges categories. For data balance, only the data of the fifth day is selected as a sample for normal traffic (see Table 1).
[0154] Table 1
[0155]
[0156]
[0157] For data processing, it is stratified into training set and test set according...
PUM
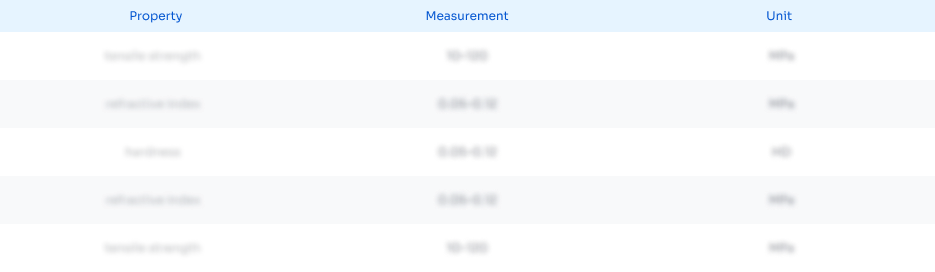
Abstract
Description
Claims
Application Information
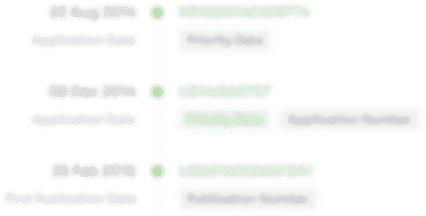
- R&D Engineer
- R&D Manager
- IP Professional
- Industry Leading Data Capabilities
- Powerful AI technology
- Patent DNA Extraction
Browse by: Latest US Patents, China's latest patents, Technical Efficacy Thesaurus, Application Domain, Technology Topic, Popular Technical Reports.
© 2024 PatSnap. All rights reserved.Legal|Privacy policy|Modern Slavery Act Transparency Statement|Sitemap|About US| Contact US: help@patsnap.com