SSVEP multi-scale noise transfer and characteristic frequency detection method based on FHN-CCA fusion
A FHN-CCA, characteristic frequency technology, applied in the field of neural engineering and brain-computer interface, can solve the original signal and EEG template correlation error, affect the detection sensitivity and recognition accuracy, and reduce the recognition accuracy rate. Correct rate and information transfer rate, avoid filter edge effects, avoid useful signal damage effects
- Summary
- Abstract
- Description
- Claims
- Application Information
AI Technical Summary
Problems solved by technology
Method used
Image
Examples
Embodiment Construction
[0040] The present invention will be further described in detail below in conjunction with the embodiments and accompanying drawings.
[0041] refer to figure 1 , a SSVEP multi-scale noise transfer and eigenfrequency detection method based on FHN-CCA fusion, including the following steps:
[0042] 1) Multi-channel data acquisition: refer to figure 2 , looking at the checkerboard movement expansion-contraction paradigm, the subjects were collected multi-channel SSVEP signals through the g.USBamp (g.tec Inc., Austria) EEG acquisition system, and the electrodes were set according to the 10 / 20 electrode system, and the SSVEP acquisition During the process, the reference electrode was located on the forehead of the brain (FPz), the ground electrode was located on the left earlobe (A1), and a total of 8 electrodes, POz, PO3, PO4, PO5, PO6, Oz, O1 and O2, were used to record SSVEP signals, and the SSVEP signals were recorded at a frequency of 1000 Hz Sampling, filtered by a 50Hz n...
PUM
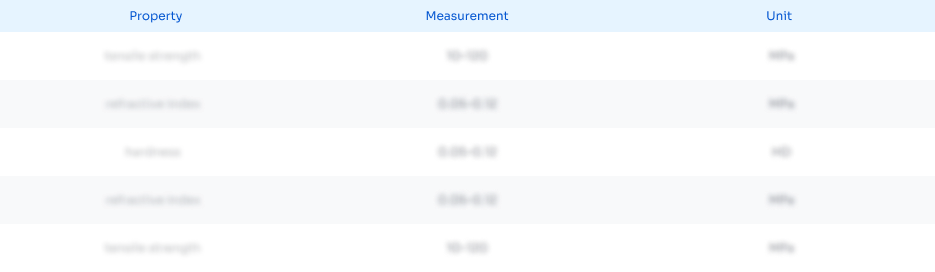
Abstract
Description
Claims
Application Information
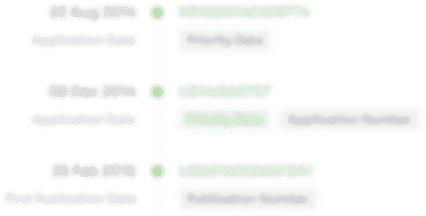
- R&D Engineer
- R&D Manager
- IP Professional
- Industry Leading Data Capabilities
- Powerful AI technology
- Patent DNA Extraction
Browse by: Latest US Patents, China's latest patents, Technical Efficacy Thesaurus, Application Domain, Technology Topic, Popular Technical Reports.
© 2024 PatSnap. All rights reserved.Legal|Privacy policy|Modern Slavery Act Transparency Statement|Sitemap|About US| Contact US: help@patsnap.com