Method for establishing early screening light-chain amyloidosis based on machine learning and application thereof
An amyloidosis and machine learning technology, applied in the fields of instrumentation, informatics, medical informatics, etc., to improve the early diagnosis rate, easy to popularize and use, and improve the accuracy.
- Summary
- Abstract
- Description
- Claims
- Application Information
AI Technical Summary
Problems solved by technology
Method used
Image
Examples
Embodiment 1
[0049] Example 1 Establishment of a method for screening light chain amyloidosis based on machine learning
[0050] 1. The random forest model is an integrated learning algorithm based on decision trees, and its construction process is as follows:
[0051] (1) Assuming that there are N samples, then randomly select n samples with replacement; use the selected n samples to train a decision tree as the sample at the root node of the decision tree;
[0052] (2) When each sample has M attributes, when each node of the decision tree needs to be split, randomly select m attributes from the M attributes, satisfying the condition m
[0053] where p i,k is the proportion of training instances of class k on the i-th node;
[0054] (3) During the formation of the decision tree, each node must be split according to step 2 until it can no longer be split;
[0055] (4) Build a large number of decision trees according to steps 1 to 3, thus forming a random forest.
[0056] 2. The suppo...
Embodiment 2
[0078] Example 2 The clinical application of a method for early screening of light chain amyloidosis based on machine learning.
[0079] The Medical Ethics Committee of Shengjing Hospital of China Medical University approved this study (2020PS055J) according to the principles of the Declaration of Helsinki. After the approval of the ethics committee and the exemption of informed consent, the patient data were anonymized and analyzed retrospectively. The flowchart and complete model training process as figure 1 shown.
[0080] 1. Patients and Data Sources
[0081] From January 2010 to January 2020, the relevant data of 1064 patients were collected in 18 hospitals in the China Light Chain Amyloidosis Registry Network (CRENLA), including general information, blood routine, urine test, biochemical test and echocardiography. In addition, the relevant data of 1000 cases of non-AL amyloidosis (heart failure, cardiomyopathy, liver disease and kidney disease) were randomly selected...
PUM
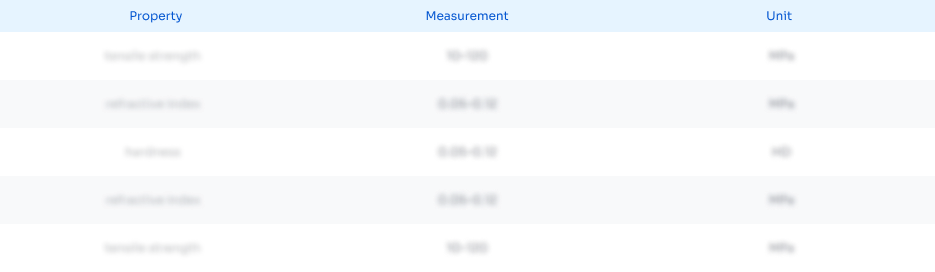
Abstract
Description
Claims
Application Information
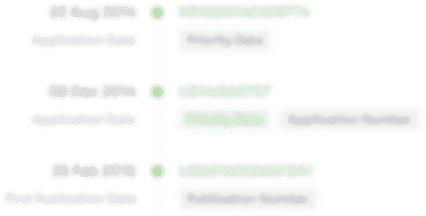
- R&D Engineer
- R&D Manager
- IP Professional
- Industry Leading Data Capabilities
- Powerful AI technology
- Patent DNA Extraction
Browse by: Latest US Patents, China's latest patents, Technical Efficacy Thesaurus, Application Domain, Technology Topic, Popular Technical Reports.
© 2024 PatSnap. All rights reserved.Legal|Privacy policy|Modern Slavery Act Transparency Statement|Sitemap|About US| Contact US: help@patsnap.com