Foundation pit disaster prediction and early warning method based on LSTM and deep residual neural network
A neural network and foundation pit technology, applied in neural learning methods, biological neural network models, neural architectures, etc., can solve the problem of low accuracy in processing time-related data, the accuracy rate has not improved too much, and the training model time is increased To achieve the effect of improving the prediction of foundation pit monitoring data, increasing the probability and possibility, and avoiding foundation pit disasters
- Summary
- Abstract
- Description
- Claims
- Application Information
AI Technical Summary
Problems solved by technology
Method used
Image
Examples
Embodiment Construction
[0066] In order to make the object, technical solution and advantages of the present invention clearer, the implementation manner of the present invention will be further described in detail below in conjunction with the accompanying drawings.
[0067] The present invention provides a foundation pit disaster prediction and early warning method based on LSTM and deep residual neural network, please refer to Figure 1-10 , including the following steps:
[0068] S1, at the key points of the foundation pit to be measured, place measuring points for measuring the data of groundwater level, supporting axial force, ground connection wall stress and deep horizontal displacement at the point;
[0069] S2, collecting the above data, and preprocessing it;
[0070] S3, constructing LSTM four-layer neural network for groundwater level data;
[0071] S4, constructing LSTM two-layer + GRU two-layer neural network for the supporting axial force data;
[0072] S5, constructing LSTM three-l...
PUM
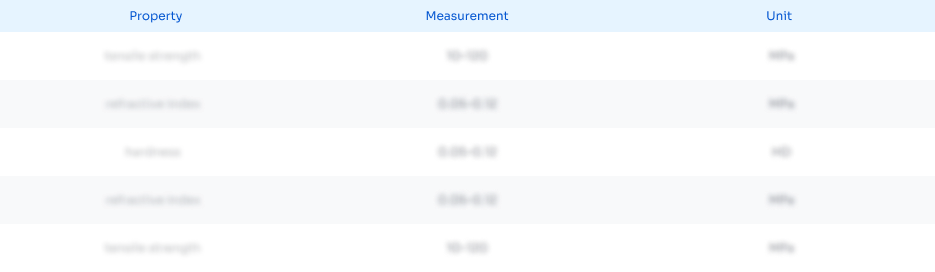
Abstract
Description
Claims
Application Information
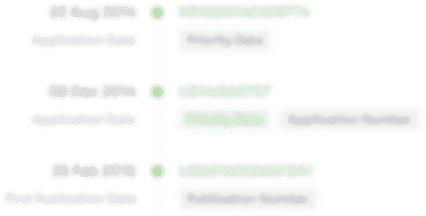
- R&D Engineer
- R&D Manager
- IP Professional
- Industry Leading Data Capabilities
- Powerful AI technology
- Patent DNA Extraction
Browse by: Latest US Patents, China's latest patents, Technical Efficacy Thesaurus, Application Domain, Technology Topic, Popular Technical Reports.
© 2024 PatSnap. All rights reserved.Legal|Privacy policy|Modern Slavery Act Transparency Statement|Sitemap|About US| Contact US: help@patsnap.com