Air temperature forecast data correction method based on deep learning
A technology for forecasting data and deep learning, applied in neural learning methods, pattern recognition in signals, instruments, etc. Resolution, the effect of improving spatiotemporal resolution
- Summary
- Abstract
- Description
- Claims
- Application Information
AI Technical Summary
Problems solved by technology
Method used
Image
Examples
Embodiment Construction
[0025] The scheme of the present invention is further described below:
[0026] Detailed steps of the present invention are:
[0027] S1. Acquiring original weather forecast data and historical weather observation data, the meteorological elements included in the original weather forecast data and historical weather observation data need to have air temperature elements and at least one other meteorological element characteristics related to air temperature elements;
[0028] S2. Preprocessing the data obtained in step S1, including:
[0029] S21, performing missing value processing and abnormal value processing on the historical meteorological observation data;
[0030] Missing value processing: determine whether there are missing values in the temperature meteorological elements and other related meteorological features within the preset time period, and count the number of missing values. When the number of missing values is less than the set value and the two data bef...
PUM
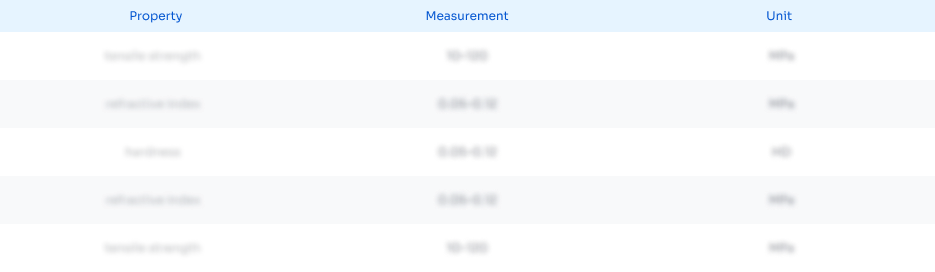
Abstract
Description
Claims
Application Information
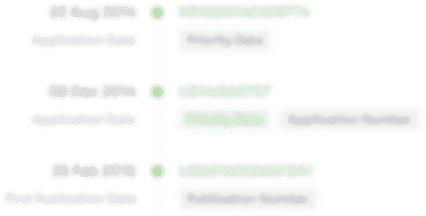
- Generate Ideas
- Intellectual Property
- Life Sciences
- Materials
- Tech Scout
- Unparalleled Data Quality
- Higher Quality Content
- 60% Fewer Hallucinations
Browse by: Latest US Patents, China's latest patents, Technical Efficacy Thesaurus, Application Domain, Technology Topic, Popular Technical Reports.
© 2025 PatSnap. All rights reserved.Legal|Privacy policy|Modern Slavery Act Transparency Statement|Sitemap|About US| Contact US: help@patsnap.com