Method for predicting relapse of cerebral arterial thrombosis
A technology of ischemic stroke and prediction method, which is applied in the direction of neural learning method, instrument, biological neural network model, etc., can solve the problem of unbalanced data filling sample imbalance, few recurrence prediction studies, non-imaging data and hospitalization structure to achieve the effects of high accuracy, strong mining ability, and strong extraction ability
- Summary
- Abstract
- Description
- Claims
- Application Information
AI Technical Summary
Problems solved by technology
Method used
Image
Examples
Embodiment
[0069] (1) The hospitalization records of 2,817 patients with ischemic stroke were collected from the prospective cohort of the Medical Big Data Research Center, and all of these patients were confirmed to be ischemic stroke by MRI. The data are roughly divided into: demographic information (gender, age, marriage), inpatient laboratory test data, structured data after inpatient electronic medical records, and imaging data.
[0070] (2) Analyzing the distribution of all samples in the follow-up records of the following year, 326 relapsed and 2491 non-relapsed, the relapse rate was about 13.08%, and there was an extreme sample imbalance. There are 163 people in the non-relapse group who have previous hospitalization information, so the latest hospitalization information is used to fill in the blank value of this part of patients.
[0071] (3) Sequentially calculate the correlation between the data structured features and whether the dependent variable recurs, and select the feat...
PUM
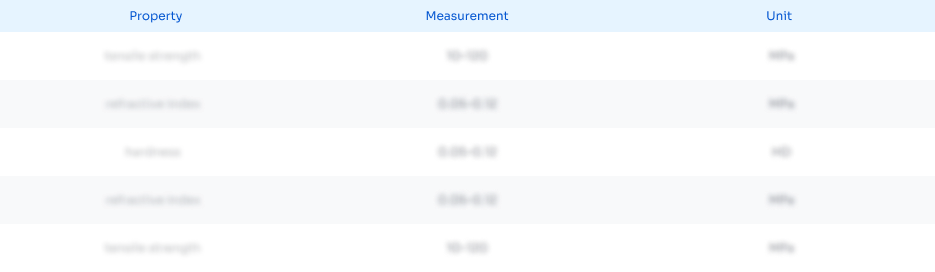
Abstract
Description
Claims
Application Information
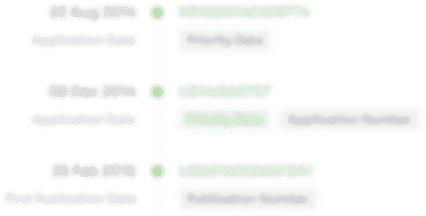
- R&D Engineer
- R&D Manager
- IP Professional
- Industry Leading Data Capabilities
- Powerful AI technology
- Patent DNA Extraction
Browse by: Latest US Patents, China's latest patents, Technical Efficacy Thesaurus, Application Domain, Technology Topic, Popular Technical Reports.
© 2024 PatSnap. All rights reserved.Legal|Privacy policy|Modern Slavery Act Transparency Statement|Sitemap|About US| Contact US: help@patsnap.com