Neural network acceleration hardware architecture and method for quantization bit width dynamic selection
A neural network and hardware architecture technology, applied in neural architecture, neural learning methods, biological neural network models, etc., can solve the problem of not taking into account the differences of neurons, and achieve the effect of reducing inference time, ensuring accuracy, and improving performance
- Summary
- Abstract
- Description
- Claims
- Application Information
AI Technical Summary
Problems solved by technology
Method used
Image
Examples
Embodiment Construction
[0044] To achieve the purpose of the present invention, the present invention comprises the following operating steps:
[0045] Step 1. According to the parallel computing characteristics of the deployed hardware, the feature map in the network is divided into blocks as units of neurons. In each block, continue to be divided into groups in the spatial dimension, and the group is defined as the dynamic quantization operation. smallest unit.
[0046] Step 2. Configure a trainable threshold parameter for each block in all feature maps of the target network, and determine the upper and lower bounds of the selectable sparsity of each block according to the given basic quantization bit width and the total amount of target bit width constraints .
[0047] Step 3. Establish a dynamic quantified neural network training and inference model, divide the inference into two parts: high-precision and low-precision calculations, and judge whether to perform low-precision calculations based on ...
PUM
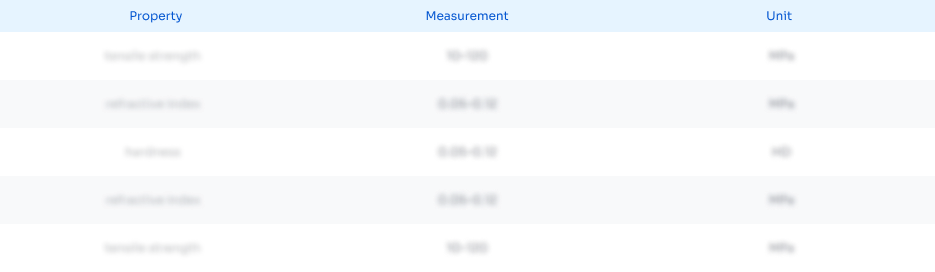
Abstract
Description
Claims
Application Information
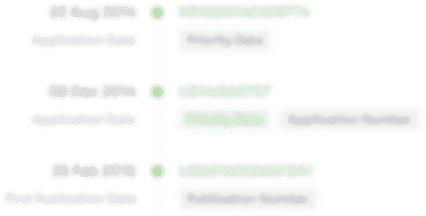
- R&D
- Intellectual Property
- Life Sciences
- Materials
- Tech Scout
- Unparalleled Data Quality
- Higher Quality Content
- 60% Fewer Hallucinations
Browse by: Latest US Patents, China's latest patents, Technical Efficacy Thesaurus, Application Domain, Technology Topic, Popular Technical Reports.
© 2025 PatSnap. All rights reserved.Legal|Privacy policy|Modern Slavery Act Transparency Statement|Sitemap|About US| Contact US: help@patsnap.com