Group discovery method based on depth map convolutional network
A technology of convolutional network and discovery method, applied in the field of group discovery based on deep graph convolutional network, which can solve the problem that node labels cannot use global network information, it is difficult to obtain a large number of a priori labeled nodes, model structure and scalability are insufficient and other problems to achieve the effect of avoiding excessive smoothing problems, improving accuracy, and strong practicability
- Summary
- Abstract
- Description
- Claims
- Application Information
AI Technical Summary
Problems solved by technology
Method used
Image
Examples
Embodiment Construction
[0053] The present invention will be described in detail below in conjunction with specific embodiments. The following examples will help those skilled in the art to further understand the present invention, but do not limit the present invention in any form. It should be noted that those skilled in the art can make several changes and improvements without departing from the concept of the present invention. These all belong to the protection scope of the present invention.
[0054] The present invention is described in further detail below in conjunction with accompanying drawing:
[0055] See attached Figure 1-4 , an embodiment of the present invention provides a group discovery method for a deep graph convolutional network. In view of the dependence of previous methods on a large amount of labeled data and the problem that the network structure cannot be deepened due to over-smoothing, this method proposes a weakly supervised learning model that combines pre-training an...
PUM
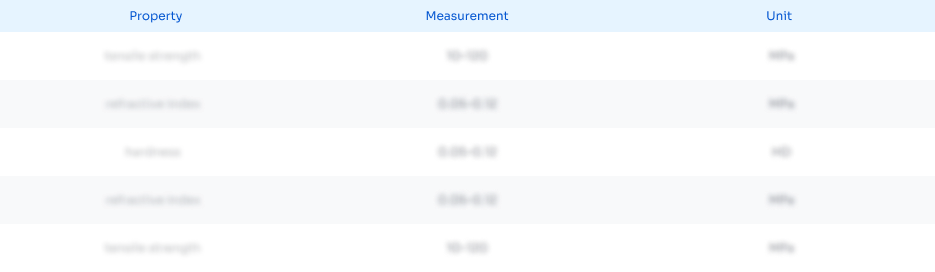
Abstract
Description
Claims
Application Information
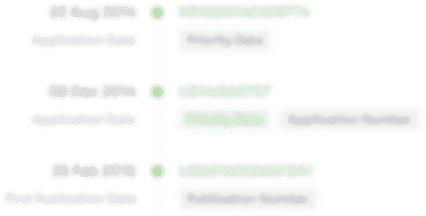
- R&D
- Intellectual Property
- Life Sciences
- Materials
- Tech Scout
- Unparalleled Data Quality
- Higher Quality Content
- 60% Fewer Hallucinations
Browse by: Latest US Patents, China's latest patents, Technical Efficacy Thesaurus, Application Domain, Technology Topic, Popular Technical Reports.
© 2025 PatSnap. All rights reserved.Legal|Privacy policy|Modern Slavery Act Transparency Statement|Sitemap|About US| Contact US: help@patsnap.com