A Drug-Target Interaction Prediction Method Based on Contrastive Learning of Supervised Synergy Graphs
A prediction method and supervised technology, applied in the field of drug-target relationship prediction, can solve the problems of too many complicated steps in the model and tedious feature extraction, and achieve the effect of enhancing learning ability, reducing processing steps, and high prediction accuracy.
- Summary
- Abstract
- Description
- Claims
- Application Information
AI Technical Summary
Problems solved by technology
Method used
Image
Examples
specific Embodiment approach 1
[0046] Specific implementation mode 1. Combination figure 1 This embodiment will be described. A method for predicting drug-target interaction based on supervised synergy graph comparative learning described in this embodiment specifically includes the following steps:
[0047] Step S1, extracting drug information, protein information, disease information and drug side effect information from the database, and constructing a drug heterogeneous information network and a protein heterogeneous information network according to the extracted information;
[0048] Step S2, obtaining the final representation of each drug in the drug heterogeneous information network based on the first encoder, and obtaining the final representation of each protein in the protein heterogeneous information network based on the second encoder;
[0049] The specific process of obtaining the final representation of each drug in the drug heterogeneous information network based on the first encoder is as f...
specific Embodiment approach 2
[0063] Embodiment 2: The difference between this embodiment and Embodiment 1 is that the specific process of step S1 is:
[0064] Extracting drug information from the DrugBank database, the drug information includes drug-drug interaction information and known drug-protein interaction information;
[0065] Extract protein information from the HPRD database, where the protein information is protein-protein interaction information;
[0066] extracting disease information from a toxicogenomics database, the disease information including disease-drug relationship information and disease-protein relationship information;
[0067] Extract drug side effect information from the SIDER database, where the drug side effect information is the relationship information between drugs and side effects;
[0068] Construct a drug heterogeneous information network according to the interaction information between drugs, the interaction information between drugs and proteins, the relationship info...
specific Embodiment approach 3
[0074] Embodiment 3: The difference between this embodiment and Embodiment 1 or 2 is that the attention mechanism is used to give different weights to different meta-paths, and the representations of each meta-path are weighted and summed according to the weights, and the weighted and the result as the final representation of the drug corresponding to this node; it is specifically:
[0075]
[0076] in, is the representation of the i-th metapath output by the first encoder, W is the weight matrix, b is the bias vector, q is the transformation vector, is the weight given to the i-th meta-path;
[0077] Weighted summation of the representations of individual metapaths:
[0078]
[0079] where h drug is the final representation of the drug corresponding to this node, i=1,2,...M d , M d is the total number of all meta-paths including this node in the drug heterogeneous information network.
[0080] Other steps and parameters are the same as in the first or second emb...
PUM
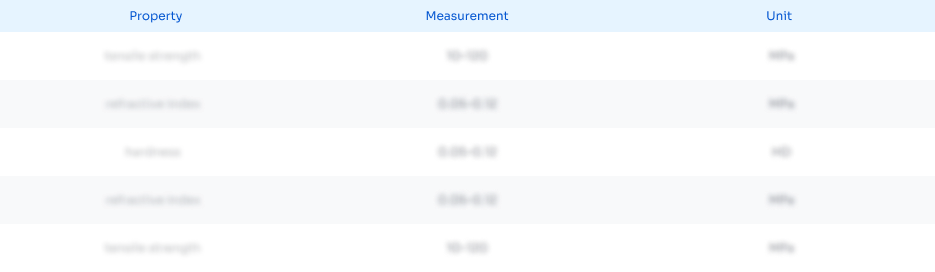
Abstract
Description
Claims
Application Information
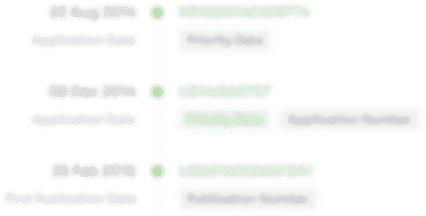
- R&D Engineer
- R&D Manager
- IP Professional
- Industry Leading Data Capabilities
- Powerful AI technology
- Patent DNA Extraction
Browse by: Latest US Patents, China's latest patents, Technical Efficacy Thesaurus, Application Domain, Technology Topic, Popular Technical Reports.
© 2024 PatSnap. All rights reserved.Legal|Privacy policy|Modern Slavery Act Transparency Statement|Sitemap|About US| Contact US: help@patsnap.com