Short-term power load prediction method, computer equipment and storage medium
A short-term power load and power load technology, applied in the field of power engineering, can solve the problems of inaccurate feature extraction of meteorological factors and low prediction accuracy, and achieve the effect of improving globality and integrity and improving accuracy.
- Summary
- Abstract
- Description
- Claims
- Application Information
AI Technical Summary
Problems solved by technology
Method used
Image
Examples
Embodiment 1
[0056] Such as Figure 1 to Figure 10 As shown, a short-term power load forecasting method using KNN and BiLSTM includes the following steps:
[0057] S1: Obtain weather data and power load data as sample data;
[0058] S2: Divide the sample data into training set and test set after cleaning and normalizing;
[0059] S3: Use the KNN algorithm to sort the sample data from large to small and obtain the eigenvalue K;
[0060] S4: Select all the weather factors contained in the first K eigenvalues as input terminals for prediction;
[0061] S5: Input all weather factor data and historical power load data contained in the first K eigenvalues as the training data for this prediction;
[0062] S6: Use BiLSTM to establish a power load forecasting model and adjust BiLSTM hyperparameters;
[0063] S7: Use mean absolute error (MAE), mean absolute percentage error (MAPE), root mean square error (RMSE) and coefficient of determination R^2 to carry out detailed comparative analysis ...
Embodiment 2
[0189] This embodiment provides a computer device, including at least one processor and a memory; the memory stores computer-executable instructions; the at least one processor executes the computer-executable instructions stored in the memory, so that the at least one processor executes The short-term power load forecasting method using KNN and BiLSTM described in Embodiment 1.
Embodiment 3
[0191]This embodiment provides a computer-readable storage medium, where computer-executable instructions are stored in the computer-readable storage medium, and when the processor executes the computer-executable instructions, the implementation of KNN and BiLSTM described in Embodiment 1 is realized. Short-term electric load forecasting method.
PUM
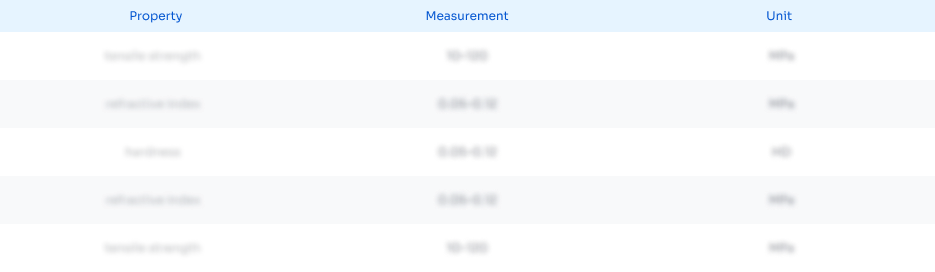
Abstract
Description
Claims
Application Information
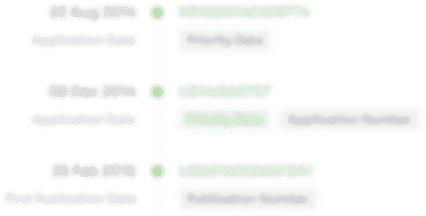
- R&D
- Intellectual Property
- Life Sciences
- Materials
- Tech Scout
- Unparalleled Data Quality
- Higher Quality Content
- 60% Fewer Hallucinations
Browse by: Latest US Patents, China's latest patents, Technical Efficacy Thesaurus, Application Domain, Technology Topic, Popular Technical Reports.
© 2025 PatSnap. All rights reserved.Legal|Privacy policy|Modern Slavery Act Transparency Statement|Sitemap|About US| Contact US: help@patsnap.com