Multi-modal retrieval method and system based on weak supervision hash learning
A weakly supervised, multi-modal technology, applied in the field of big data retrieval, can solve complex optimization problems, do not solve the semantic gap and other problems, achieve the effect of improving performance, bridging the cross-modal semantic gap, and improving retrieval accuracy
- Summary
- Abstract
- Description
- Claims
- Application Information
AI Technical Summary
Problems solved by technology
Method used
Image
Examples
Embodiment 1
[0044] refer to figure 1 with figure 2 , the present embodiment provides a multimodal retrieval method based on weakly supervised hash learning, which specifically includes the following steps:
[0045] S101: Obtain samples to be retrieved, and perform hash code calculation on them.
[0046]For image samples , generating its hash code ; for text samples , generating its hash code ; Among them, the sign function is a quantization function, the purpose is to quantize the real value output by the network into a discrete 0 / 1 code, which is convenient for subsequent retrieval.
[0047] S102: Perform a 0 / 1 XOR operation on the hash code of the sample to be retrieved and the hash code in the retrieval database to calculate the Hamming distance, and return similar data in order of the Hamming distance from small to large.
[0048] What needs to be explained here is that the construction of the retrieval database is constructed in an offline manner.
[0049] In the specific...
Embodiment 2
[0088] Such as image 3 As shown, the present embodiment provides a multimodal retrieval system based on weakly supervised hash learning, which specifically includes the following modules:
[0089] (1) Hash code calculation module, which is used to obtain samples to be retrieved and perform hash code calculation on them;
[0090] (2) Online retrieval module, which is used to perform 0 / 1 XOR operation between the hash code of the sample to be retrieved and the hash code in the retrieval database, calculate the Hamming distance, and return the similarity according to the Hamming distance from small to large data;
[0091] Wherein, the construction process of the retrieval database is:
[0092] Based on the intra-modal pairwise similarity, the inter-modal pairwise similarity and the completed label information of each modality, the objective function of semi-supervised semi-pair cross-modal hashing is established;
[0093] Obtain hash representation by optimizing the objective f...
Embodiment 3
[0103] This embodiment provides a computer-readable storage medium, on which a computer program is stored. When the program is executed by a processor, the steps in the multimodal retrieval method based on weakly supervised hash learning as described above are implemented.
PUM
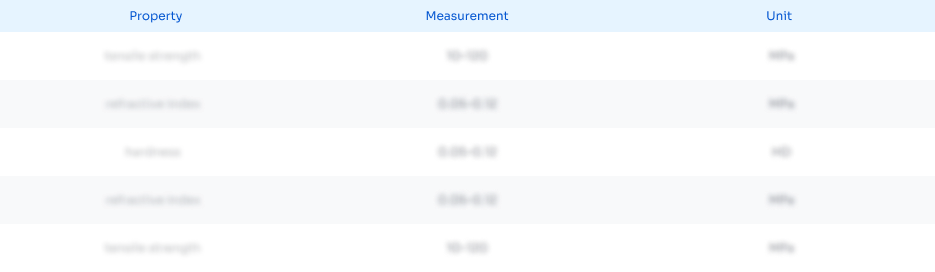
Abstract
Description
Claims
Application Information
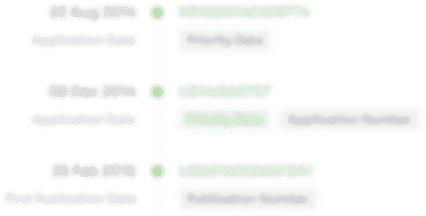
- R&D Engineer
- R&D Manager
- IP Professional
- Industry Leading Data Capabilities
- Powerful AI technology
- Patent DNA Extraction
Browse by: Latest US Patents, China's latest patents, Technical Efficacy Thesaurus, Application Domain, Technology Topic, Popular Technical Reports.
© 2024 PatSnap. All rights reserved.Legal|Privacy policy|Modern Slavery Act Transparency Statement|Sitemap|About US| Contact US: help@patsnap.com