High-water-cut-period oil reservoir oil well yield prediction method based on machine learning
A prediction method and technology of high water-cut period, applied in nuclear methods, surveying, earthwork drilling, etc., can solve the problems of many factors, large amount of calculation, complicated steps, etc. Effect
- Summary
- Abstract
- Description
- Claims
- Application Information
AI Technical Summary
Problems solved by technology
Method used
Image
Examples
Embodiment 1
[0043] Example 1 A method for predicting oil well production in high water-cut oil reservoirs based on machine learning
[0044] The method includes:
[0045] Step 1. Judge the target reservoir and select the appropriate block:
[0046] The method of judging the target oil reservoir is: judging the correlation between the water injection well and the oil production well in the target oil reservoir, select the injection production well with high correlation, draw the production curve of the production well and the water injection curve of the water injection well, and judge the water injection well according to the two curves. Whether there is a co-increase and co-decrease between the water injection amount and the production of the oil production well, if so, the oil reservoir is a suitable oil reservoir.
[0047] Step 2. Detect the data stability of the water injection curve of the water injection well and the production curve of the oil production well respectively. If the ...
Embodiment 2
[0062] Example 2 A method for predicting oil well production in high water-cut oil reservoirs based on machine learning
[0063] Taking oil and water wells in an actual block in China as an example, the method for predicting oil well production in high water-cut oil reservoirs based on machine learning specifically includes:
[0064] A time series curve is drawn for the production data of oil and water wells in this block. The production date of oil and water wells in this block is from March 1969 to December 2018. Draw the co-correlation matrix of water injection wells and oil production wells in this block, such as figure 1 As shown, the brighter the color block, the higher the correlation of production curves between injection and production wells, and the darker the color block, the lower. It can be seen that there is a good correlation between some injection and production wells in this block, and the production curve of Xi 46-6-1 oil production well and the water inject...
PUM
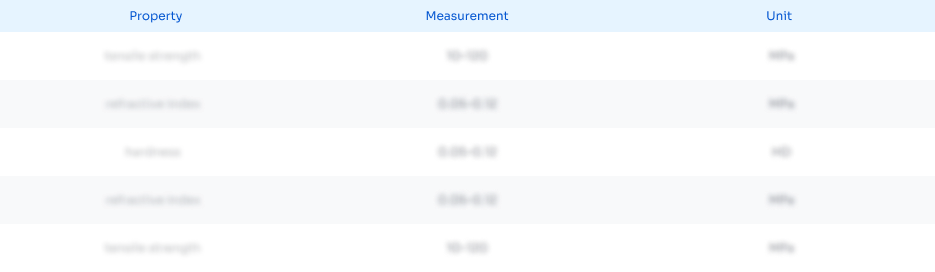
Abstract
Description
Claims
Application Information
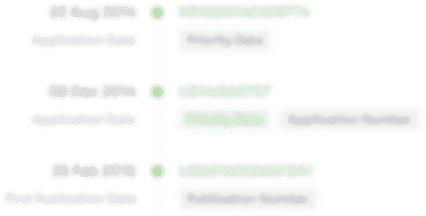
- Generate Ideas
- Intellectual Property
- Life Sciences
- Materials
- Tech Scout
- Unparalleled Data Quality
- Higher Quality Content
- 60% Fewer Hallucinations
Browse by: Latest US Patents, China's latest patents, Technical Efficacy Thesaurus, Application Domain, Technology Topic, Popular Technical Reports.
© 2025 PatSnap. All rights reserved.Legal|Privacy policy|Modern Slavery Act Transparency Statement|Sitemap|About US| Contact US: help@patsnap.com