Intelligent video image retrieval method based on neural network self-temperature fault and knowledge conduction mechanism
A neural network and video image technology, used in video data retrieval, neural learning methods, biological neural network models, etc., can solve the problems of uneven illumination robustness, poor real-time performance, etc. Accuracy, the effect of improving retrieval accuracy
- Summary
- Abstract
- Description
- Claims
- Application Information
AI Technical Summary
Problems solved by technology
Method used
Image
Examples
Embodiment Construction
[0080] The technical solutions of the present invention will be further described in detail below with reference to the accompanying drawings.
[0081] The invention proposes an image retrieval method based on neural network self-review and knowledge transfer mechanism, and aims to realize a retrieval model with simple, high efficiency, high precision, good generalization and strong robustness. The algorithm includes the following parts: 1. Image correction module; 2. Feature extraction module; 3. Conduction module; 4. Self-examination module, such as figure 1 shown.
[0082] Due to the influence of factors such as the underground environment and lighting equipment, the image is affected by uneven illumination, which is easy to cause retrieval errors. Therefore, the present invention proposes a gamma correction module to achieve the purpose of reconstructing image lighting conditions. Different from the traditional bilinear filtering, median filtering, histogram homogenizati...
PUM
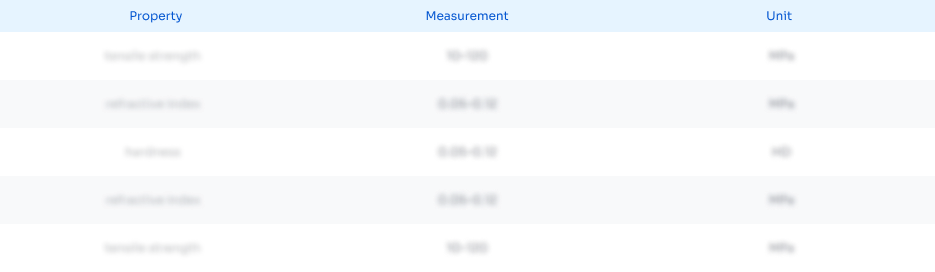
Abstract
Description
Claims
Application Information
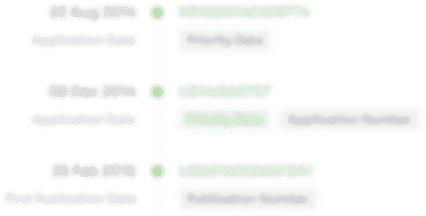
- R&D Engineer
- R&D Manager
- IP Professional
- Industry Leading Data Capabilities
- Powerful AI technology
- Patent DNA Extraction
Browse by: Latest US Patents, China's latest patents, Technical Efficacy Thesaurus, Application Domain, Technology Topic.
© 2024 PatSnap. All rights reserved.Legal|Privacy policy|Modern Slavery Act Transparency Statement|Sitemap