Depth unsupervised single cell clustering method based on Gaussian mixture graph variational auto-encoder
A Gaussian mixture, autoencoder technology, applied in neural learning methods, instruments, genomics, etc., can solve problems such as lack of single peak judgment, wrong definition, and neglect of interaction relationships
- Summary
- Abstract
- Description
- Claims
- Application Information
AI Technical Summary
Problems solved by technology
Method used
Image
Examples
Embodiment Construction
[0063] The present invention will be further described in detail below with reference to the accompanying drawings and specific embodiments. It should be understood that the specific embodiments described herein are only used to explain the present invention, but not to limit the present invention.
[0064] like figure 1 As shown, the first aspect of the embodiment of the present invention provides a deep unsupervised single-cell clustering method based on Gaussian mixture graph variational autoencoder (VGAE), which is obtained by using a graph variational autoencoder based on Gaussian mixture distribution. A method for gene regulation network A, cell low-dimensional representation Z and cell clustering C, including the following steps:
[0065] Step 1: Input protein-protein interaction relationship PPIs, single-cell gene expression data X;
[0066] Step 2: Initialize trainable gene regulation network A using PPIs, the specific steps are as follows:
[0067] Step 2.1: Numbe...
PUM
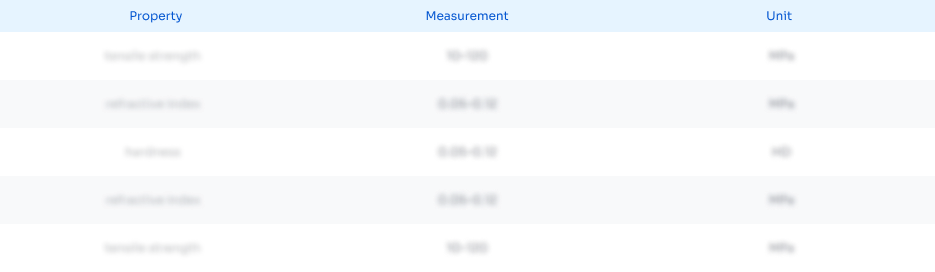
Abstract
Description
Claims
Application Information
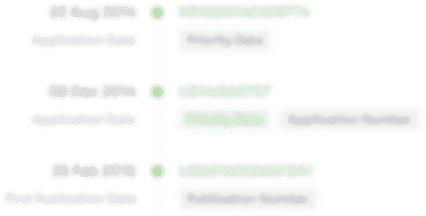
- R&D Engineer
- R&D Manager
- IP Professional
- Industry Leading Data Capabilities
- Powerful AI technology
- Patent DNA Extraction
Browse by: Latest US Patents, China's latest patents, Technical Efficacy Thesaurus, Application Domain, Technology Topic, Popular Technical Reports.
© 2024 PatSnap. All rights reserved.Legal|Privacy policy|Modern Slavery Act Transparency Statement|Sitemap|About US| Contact US: help@patsnap.com