Motor fault detection method based on group type sparse self-coding and swarm intelligence
A sparse autoencoder and sparse autoencoder technology, which is applied in the direction of motor generator testing, electrical winding testing, neural learning methods, etc., can solve problems such as indistinct fault characteristic signals, excessive reliance on prior knowledge, and generalization ability constraints , to achieve the effect of eliminating the necessity of manually selecting features, realizing fine diagnosis, and solving complexity
- Summary
- Abstract
- Description
- Claims
- Application Information
AI Technical Summary
Problems solved by technology
Method used
Image
Examples
Embodiment Construction
[0074] The present invention will be further described below in conjunction with the accompanying drawings. The following examples are only used to illustrate the technical solutions of the present invention more clearly, and cannot be used to limit the protection scope of the present invention.
[0075] The invention proposes a motor fault detection method based on group-type sparse auto-encoder and group intelligence. By establishing a group-type sparse auto-encoder (GSAE) implementation framework, and using the M-M method to solve the problem, the original input motor is realized. Feature extraction of fault data; proposed particle swarm algorithm (SS-PSO) integrating self and social factors; established SS-PSO-ANN deep classifier model; used SS-PSO-ANN classifier to effectively diagnose motor faults. The invention can automatically learn the intrinsic characteristics of the motor fault data, mine the deep-level abstract characteristics of the data and use them as the chara...
PUM
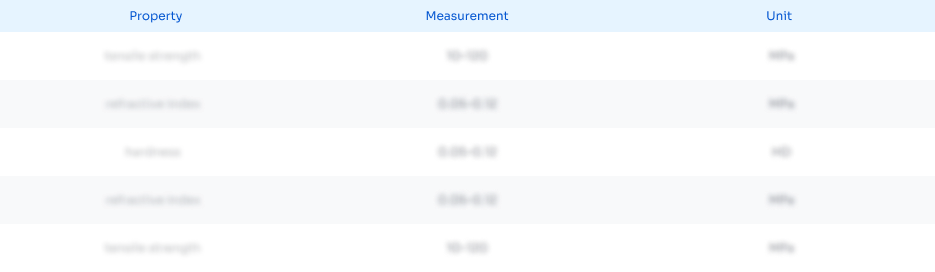
Abstract
Description
Claims
Application Information
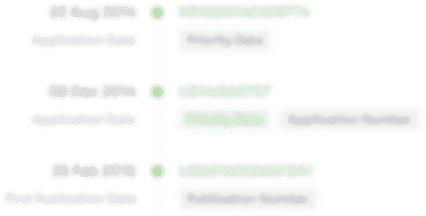
- R&D Engineer
- R&D Manager
- IP Professional
- Industry Leading Data Capabilities
- Powerful AI technology
- Patent DNA Extraction
Browse by: Latest US Patents, China's latest patents, Technical Efficacy Thesaurus, Application Domain, Technology Topic, Popular Technical Reports.
© 2024 PatSnap. All rights reserved.Legal|Privacy policy|Modern Slavery Act Transparency Statement|Sitemap|About US| Contact US: help@patsnap.com