Electromyographic signal classification method based on multi-kernel support vector machine
A technology of support vector machine and electromyographic signal, which is applied in the field of pattern recognition, can solve the problems of large randomness, time-consuming, classification accuracy and the influence of the number of support vectors, etc., so as to improve classification accuracy, broad application prospects, and balance accuracy and real-time effect
- Summary
- Abstract
- Description
- Claims
- Application Information
AI Technical Summary
Problems solved by technology
Method used
Image
Examples
Embodiment Construction
[0031] The movement of the lower limbs of the human body can be divided into various movement modes such as horizontal walking, going up steps, going down steps, standing, sitting down, standing up, turning, and jogging. Considering the basic functions that the prosthesis is urgently required to achieve, this paper uses four motion modes of walking horizontally, going up steps, going down steps, and standing as examples. Therefore, the designed multi-class classifier needs to recognize four types of modes.
[0032] The implementation of the EMG signal classification method based on multi-core SVM mainly includes four steps: (1) collection of lower limb EMG signals; (2) denoising processing; (3) feature extraction; (4) based on multi-core SVM classification operation.
[0033] Each step will be described in detail below one by one.
[0034] Step 1: Acquisition of lower limb EMG signals. The lower extremity information acquisition system is designed to pick up the lower extremit...
PUM
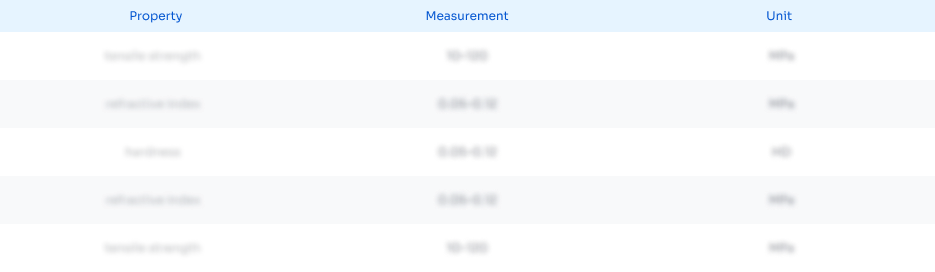
Abstract
Description
Claims
Application Information
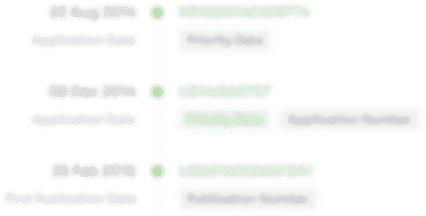
- R&D Engineer
- R&D Manager
- IP Professional
- Industry Leading Data Capabilities
- Powerful AI technology
- Patent DNA Extraction
Browse by: Latest US Patents, China's latest patents, Technical Efficacy Thesaurus, Application Domain, Technology Topic, Popular Technical Reports.
© 2024 PatSnap. All rights reserved.Legal|Privacy policy|Modern Slavery Act Transparency Statement|Sitemap|About US| Contact US: help@patsnap.com