MCSKPCA based neural network fault diagnosis method for analog circuits
A technology for simulating circuit faults and neural networks, which is applied in the fields of neural networks and electronic circuit engineering, can solve problems such as complex fault models of simulated systems, long network training time, and slow progress, and achieve easy automatic processing, short training time, and simple calculations Effect
- Summary
- Abstract
- Description
- Claims
- Application Information
AI Technical Summary
Problems solved by technology
Method used
Image
Examples
Embodiment Construction
[0057] The present invention will be described in detail below in conjunction with accompanying drawings and embodiments.
[0058] refer to figure 1 , the overall flow chart of the present invention is made of data collection step 1, wavelet transform step 2, wavelet approximation coefficient energy feature calculation step 3, feature extraction step 4 of MCSKPCA, data normalization processing step 5, and BP neural network classifier step 6. .
[0059] The data acquisition step 1 uses the data acquisition board to collect the voltage signal of the output node of the test circuit.
[0060] Wavelet transform step 2 is to use Haar wavelet to carry out wavelet transform on the collected voltage signal.
[0061] Let f(t) be the voltage output signal, ψ(t) be the Haar wavelet function, namely
[0062] The continuous Haar wavelet transform of f(t) is:
[0063] C ( a , b ) = ...
PUM
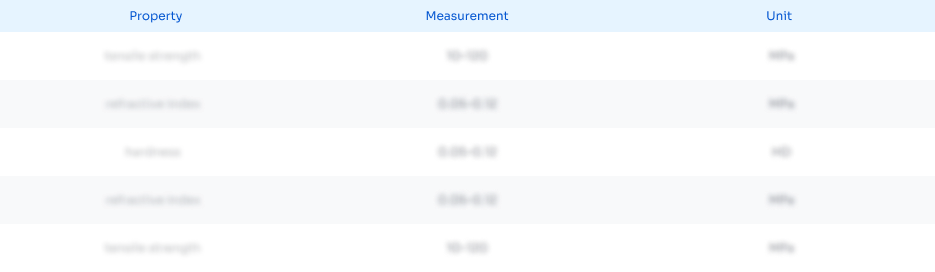
Abstract
Description
Claims
Application Information
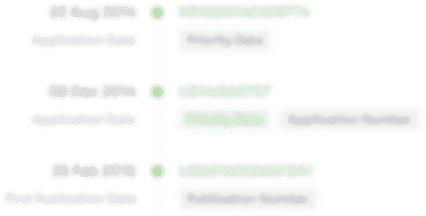
- R&D Engineer
- R&D Manager
- IP Professional
- Industry Leading Data Capabilities
- Powerful AI technology
- Patent DNA Extraction
Browse by: Latest US Patents, China's latest patents, Technical Efficacy Thesaurus, Application Domain, Technology Topic, Popular Technical Reports.
© 2024 PatSnap. All rights reserved.Legal|Privacy policy|Modern Slavery Act Transparency Statement|Sitemap|About US| Contact US: help@patsnap.com