Method for multi-channel blind deconvolution on cascaded neural network
A blind deconvolution and neural network technology, applied in the field of signal processing, can solve problems such as poor estimation of strong nonlinear functions
- Summary
- Abstract
- Description
- Claims
- Application Information
AI Technical Summary
Problems solved by technology
Method used
Image
Examples
Embodiment
[0039] In this embodiment, a method for multi-channel blind deconvolution cascaded neural network, such as figure 1 described, including the following steps:
[0040] (1) A modular neural network is composed of an equalization sub-network and a compression sub-network, the equalization sub-network is a processing unit for extracting a signal source, and the compression sub-network is a processing unit for eliminating other redundant information after extracting a signal from a mixed signal ;like figure 2 As shown, the module neural network of this embodiment is composed of an equalization sub-network and a compression sub-network. On the basis of this module neural network, the method of cascading neural networks in this embodiment can be used to obtain from unknown interleaved signals Extract multiple source signals.
[0041] (2) Balance sub-network synapse {w 1j,p} is updated by the constant model algorithm, and its update formula is as follows:
[0042] ...
PUM
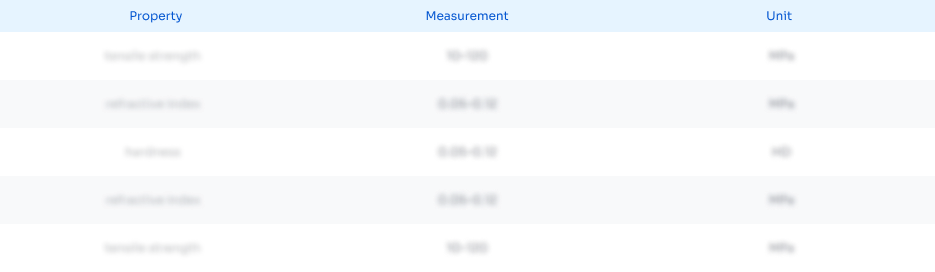
Abstract
Description
Claims
Application Information
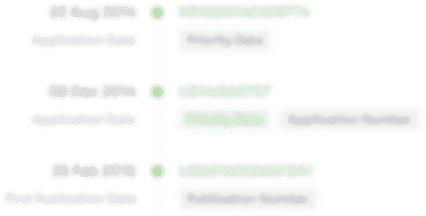
- R&D Engineer
- R&D Manager
- IP Professional
- Industry Leading Data Capabilities
- Powerful AI technology
- Patent DNA Extraction
Browse by: Latest US Patents, China's latest patents, Technical Efficacy Thesaurus, Application Domain, Technology Topic, Popular Technical Reports.
© 2024 PatSnap. All rights reserved.Legal|Privacy policy|Modern Slavery Act Transparency Statement|Sitemap|About US| Contact US: help@patsnap.com