Intrusion detection method based on observational learning
A technology of observation learning and intrusion detection, which is applied in the field of pattern recognition and machine learning, can solve the problems of not effectively using unlabeled data, algorithm performance is not necessarily the best, and the process is lengthy and boring, so as to improve the performance of classifiers and improve the overall performance, confidence-enhancing effects
- Summary
- Abstract
- Description
- Claims
- Application Information
AI Technical Summary
Problems solved by technology
Method used
Image
Examples
Embodiment 1
[0041] The invention is an intrusion detection method based on observation learning, that is, an intrusion detection method based on SSELOLA (Semi-Supervised Ensemble Learning Based Observational Learning Algorithm).
[0042] The data used in the present invention is taken from a marked data set for abnormal detection provided by DARPA for the KDD (Knowledge Discovery and Data Mining) competition in 1999, referred to as KDDCUP99. The data set includes 5 million training sets and 3 million test sets. The data includes four types of attacks: Dos (Denial of Service Attack), R2L (Unauthorized Remote Access), U2R (Illegal Access to Local Super Users) and Probe (scan and probe), the others are normal data. The present invention uses 13 of 41 attributes. Since many of these user behavior features are redundant features, only 13 of them are selected to simplify the problem.
[0043] The present invention selects all 52 pieces of data in the U2L set and marks them as the fifth category. Fr...
Embodiment 2
[0062] The method of intrusion detection based on observation learning is the same as in Example 1. Take 50% of the marked data in the training set of KDDCUP99 as an example, see figure 2 The implementation process of the intrusion detection method based on SSELOLA is as follows:
[0063] Input: Unlabeled data set x containing 147 data u , A labeled data set X containing 146 data l , A test set T containing 2959 data.
[0064] Output: the classification error rate on the test set T.
[0065] (1) Select the back-propagation neural network algorithm with hidden units of 10, 20, 30, 40 and 50 respectively, and a total of five algorithms are denoted as L 1 , L 2 , L 3 , L 4 , L 5 .
[0066] (2) For the retrieved unlabeled data set X containing 147 data u , A labeled data set containing 146 data (initial training set) X l , A test set T containing 2959 data and five learning algorithms L 1 , L 2 , L 3 , L 4 , L 5 , Combined figure 2 To X l Respectively use algorithm L 1 ~L 5 Perform train...
Embodiment 3
[0075] The method of intrusion detection based on observation and learning is the same as in Example 1-2, taking 20% of the marked data in the training set of KDDCUP99 as an example, see image 3 , The specific process is as follows:
[0076] Take 59 data into the marked data set, take 234 data into the unmarked data set, and put the remaining data into the test set. Used on the labeled data set, five back-propagation neural network learning algorithms are used for training respectively, and five classifiers are obtained. For one of the five classifiers, L, observe and learn the output of the other four classifiers in the unlabeled data set added each time, and perform majority voting based on these outputs to obtain a new labeled data set. Then add it to the training data set corresponding to the classifier, and remove the set of data in the corresponding unlabeled data set. Then use the new training data set to retrain the corresponding classifier, and then continue to obser...
PUM
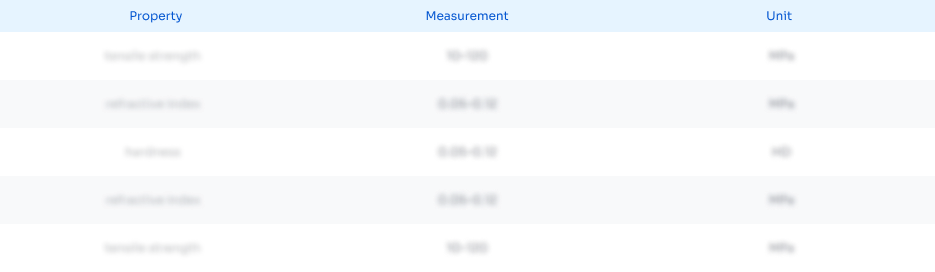
Abstract
Description
Claims
Application Information
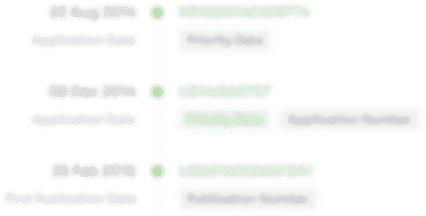
- R&D
- Intellectual Property
- Life Sciences
- Materials
- Tech Scout
- Unparalleled Data Quality
- Higher Quality Content
- 60% Fewer Hallucinations
Browse by: Latest US Patents, China's latest patents, Technical Efficacy Thesaurus, Application Domain, Technology Topic, Popular Technical Reports.
© 2025 PatSnap. All rights reserved.Legal|Privacy policy|Modern Slavery Act Transparency Statement|Sitemap|About US| Contact US: help@patsnap.com