Method for predicting thickness of hot-rolled strip steel on basis of improved partial robust M-regression algorithm
A technology of hot-rolled strip steel and regression algorithm, applied in calculation, metal rolling, measuring devices, etc., can solve problems such as time-consuming, accurate analysis model cannot be obtained, etc.
- Summary
- Abstract
- Description
- Claims
- Application Information
AI Technical Summary
Problems solved by technology
Method used
Image
Examples
specific Embodiment approach 1
[0016] Specific implementation mode one, combination figure 1 This specific embodiment is described. A hot-rolled strip thickness prediction method based on an improved partial robust M regression algorithm, which includes the following steps:
[0017] Step 1: Monitor the working data of 7 finishing mills to obtain observed variables (x i ,y i ), and according to the observed variable (x i ,y i ) Define the input data matrix X and the output data matrix Y, and calculate the initial value of the robust weighting factor ω i ;
[0018] The working data of the finishing mill includes the average distance between the work rolls of each finishing mill, the total pressure of each finishing mill, and the crimping force of the work roll of each finishing mill;
[0019] Step 2: To observe the variable (x i ,y i ) Perform weighting processing to obtain prediction data And forecast data Perform partial least squares analysis to obtain a partial least squares model of forecast data And calcul...
specific Embodiment approach 2
[0024] The second embodiment of the present embodiment is different from the first step: monitoring the working data of 7 finishing mills to obtain the observed variables (x i ,y i ), and according to the observed variable (x i ,y i ) Define the input data matrix X and the output data matrix Y, and calculate the initial value of the robust weighting factor ω i The process is:
[0025] Observed variables (x i ,y i ),among them:
[0026] x i Is the i-th row vector of input data X, x i1 ,...,x i7 Respectively is the average distance between the working rolls of each finishing mill, x i8 ,...,x i14 Respectively the total pressure of each finishing mill, x i15 ,...,x i21 Crimping force of each finishing mill work roll; y i Is the thickness of the final exported hot-rolled strip;
[0027] According to input data X and output data Y, calculate the total square loss center of input data X And the total square loss center of the output data Y
[0028] e ‾ ( X ) = X i ...
specific Embodiment approach 3
[0049] Specific embodiment three, this specific embodiment is different from specific embodiment one or two in the second step: the observation variable (x i ,y i ) Perform weighting processing to obtain prediction data And forecast data Perform partial least squares analysis to obtain a partial least squares model of forecast data Partial Least Squares Regression Model And the process of regression coefficient B is:
[0050] Do not multiply each row of the input data matrix X and the output data matrix Y by Obtain weighted observation data ( ω i x i , ω i y i ) ;
[0051] Perform partial least squares analysis on the weighted observation data to obtain the weighted least squares model:
[0052] X = TP T + X ~
[0053] Y = TQ + Y ~
[0054] Among them, T is the score matrix; P is the load matrix; Is the residual of X, and Q is the regression coefficient of the score matrix T Is the residual of Y;
[...
PUM
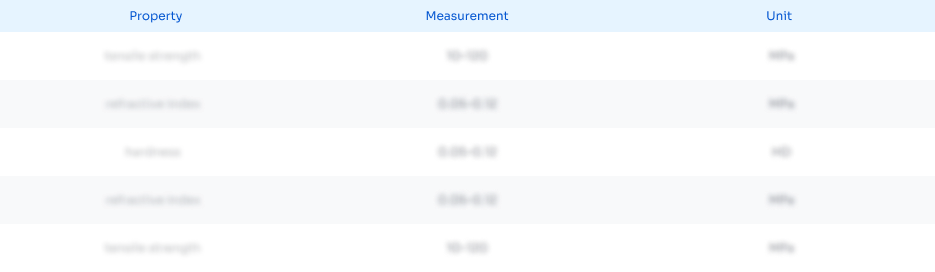
Abstract
Description
Claims
Application Information
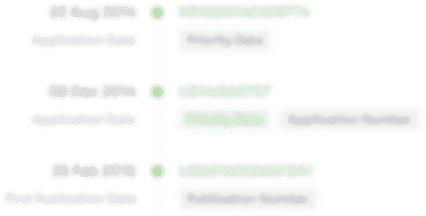
- R&D Engineer
- R&D Manager
- IP Professional
- Industry Leading Data Capabilities
- Powerful AI technology
- Patent DNA Extraction
Browse by: Latest US Patents, China's latest patents, Technical Efficacy Thesaurus, Application Domain, Technology Topic, Popular Technical Reports.
© 2024 PatSnap. All rights reserved.Legal|Privacy policy|Modern Slavery Act Transparency Statement|Sitemap|About US| Contact US: help@patsnap.com