Transformer fault detecting method based on simplified set unbalanced SVM (support vector machine)
A technology for transformer faults and detection methods, which is applied in the directions of instruments, measuring electricity, measuring devices, etc., can solve the problems of not taking into account the overall spatial structure information, and the improvement of the classification effect is not obvious.
- Summary
- Abstract
- Description
- Claims
- Application Information
AI Technical Summary
Problems solved by technology
Method used
Image
Examples
Embodiment 1
[0035] A method for detecting a fault in a transformer based on streamlined sets and reduced unbalanced SVMs, the method comprising the steps of:
[0036] (1) Use the fault feature extraction method based on the GARCH model to obtain the transformer feature vector set, and then determine the boundary samples for the minority class samples. The minority class samples are fault samples, and obtain the minority class boundary sample set ;
[0037] (2) Random selection , for collection base, , Set to 1, use the reduced set algorithm to get , repeat the operation times, where: is the number of samples in the majority class, is the number of minority class samples, so we get An artificial minority class sample, guaranteed to be at least once ;
[0038] (3) After combining the artificial minority samples generated in step (2) with the original minority samples, they are combined with the original majority sample set as the training samples of the SVM classifi...
Embodiment 2
[0042] In the described unbalanced SVM transformer fault detection method based on streamlined sets, the SVM algorithm involved and its unbalanced data classification performance analysis are as follows:
[0043] The SVM algorithm is a learning method with a strict theoretical basis. Its basic idea is to realize classification by establishing an optimal linear hyperplane, and attribute the solution of the hyperplane to solving a convex programming problem. At the same time, the Mercer kernel expansion theorem is used to upgrade the sample space to the Hilbert feature space, so that the linear learning method can be used in the feature space to solve the nonlinear classification problem of the sample space.
[0044] Taking two-class classification as an example, suppose the training sample set is , , Represents the training samples, the kernel function is . In order to prevent SVM from overfitting the training samples, it is necessary to increase the soft interval, c...
Embodiment 3
[0062] According to the simplified unbalanced SVM transformer fault detection method based on embodiment 1, the fault feature extraction method based on the GARCH model described in step (1) includes the following steps: using a vibration acceleration sensor to collect Then the vibration signal is processed by a low-pass filter with a cutoff frequency of 1500 Hz to remove high-frequency noise information and obtain a noise-reduced vibration signal; the obtained noise-reduced vibration signal is processed in sections according to time series, And use the generalized autoregressive conditional heteroscedastic model GARCH to model the segmented time series, and then select the model parameters after modeling as fault characteristics to analyze the state of the transformer. The specific method is as follows:
[0063] for vibration signals Truncate processing, get truncated signal And use GARCH (1,1) to model the truncated time series, the model is as follows:
[0064]
[00...
PUM
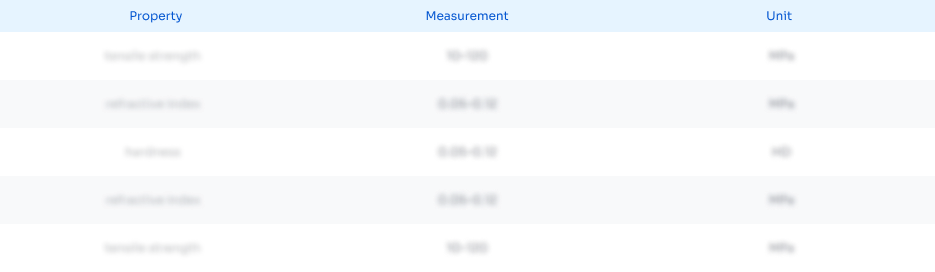
Abstract
Description
Claims
Application Information
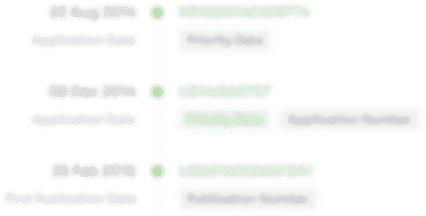
- R&D
- Intellectual Property
- Life Sciences
- Materials
- Tech Scout
- Unparalleled Data Quality
- Higher Quality Content
- 60% Fewer Hallucinations
Browse by: Latest US Patents, China's latest patents, Technical Efficacy Thesaurus, Application Domain, Technology Topic, Popular Technical Reports.
© 2025 PatSnap. All rights reserved.Legal|Privacy policy|Modern Slavery Act Transparency Statement|Sitemap|About US| Contact US: help@patsnap.com