Texture image classification method based on BoF and multi-feature fusion
A multi-feature fusion, texture image technology, applied in character and pattern recognition, instruments, computing and other directions, can solve problems such as poor description effect, and achieve the effect of improving texture description effect, good robustness, and excellent classification effect
- Summary
- Abstract
- Description
- Claims
- Application Information
AI Technical Summary
Problems solved by technology
Method used
Image
Examples
Embodiment
[0069] Such as figure 1 As shown, a flow chart of a texture image classification method based on BoF and multi-feature fusion, in order to evaluate the classification discrimination and stability of the bag of feature words obtained by the method, the embodiment simulation experiment uses the UIUC texture library, which contains 25 Each category contains 40 grayscale texture pictures of 640×480 pixels in JPG format. The experiment selects the first 30 pictures from the 6 types of wood grain and bark grain as training pictures, and the remaining 10 pictures are test pictures. figure 2 An example test image for this embodiment. Including the following steps:
[0070] Step 1: Extract the GGCM and SIFT fusion feature descriptions of Patches of each texture image in the training set, and put them into a file to form a feature set of all patches, and obtain a 143-dimensional texture feature description.
[0071] Step 1.1: SIFT feature extraction, first perform key point detection...
PUM
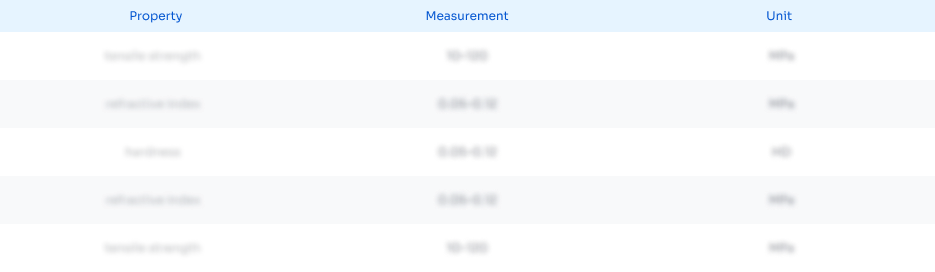
Abstract
Description
Claims
Application Information
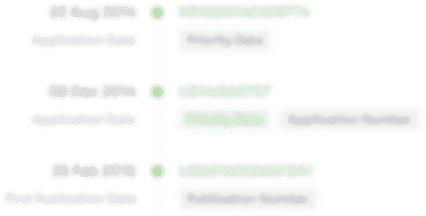
- R&D
- Intellectual Property
- Life Sciences
- Materials
- Tech Scout
- Unparalleled Data Quality
- Higher Quality Content
- 60% Fewer Hallucinations
Browse by: Latest US Patents, China's latest patents, Technical Efficacy Thesaurus, Application Domain, Technology Topic, Popular Technical Reports.
© 2025 PatSnap. All rights reserved.Legal|Privacy policy|Modern Slavery Act Transparency Statement|Sitemap|About US| Contact US: help@patsnap.com