Item-based transfer learning recommendation method and recommendation apparatus thereof
A technology of transfer learning and recommendation methods, which is applied in special data processing applications, instruments, electrical digital data processing, etc., can solve problems such as the inability to further tap learners' interests and potentials, and difficulty in meeting learners' learning requirements, so as to improve density and accuracy, save user time, and solve the effect of information overload
- Summary
- Abstract
- Description
- Claims
- Application Information
AI Technical Summary
Problems solved by technology
Method used
Image
Examples
Embodiment 1
[0041] An item-based transfer learning recommendation method, see figure 1 , the recommended method includes the following steps:
[0042] 101: Establish user-item scoring matrices for the auxiliary domain and the target domain respectively;
[0043] Carry out user modeling on the auxiliary domain and the target domain, and extract user ratings for items according to the data model of the auxiliary domain or the target domain (for example: star rating, language description, and number of views, etc.), and establish the auxiliary domain and the target domain respectively The user-item rating matrix of , where the user-item rating matrix of the auxiliary domain is denoted as R aux , and the user-item rating matrix of the target domain is denoted as R. Specifically, the number of users is denoted as n, the number of items is denoted as m, and the rating matrix R is established, then the rating value of user i on item j is denoted as Rij , where i is the user number and satisfie...
Embodiment 2
[0056] The scheme in embodiment 1 is introduced in detail below in conjunction with specific calculation formulas and examples, see the following description for details:
[0057] 201: In the process of personalized recommendation, first use the user to model the rating relationship and rating value of the item, and establish the user-item rating matrix R of the auxiliary domain through user modeling aux , the user-item rating matrix R of the target domain;
[0058] The number of users is denoted as n, the number of items is denoted as m, then the rating value of user i on item j is denoted as R ij or (R aux ) ij , where i is the user number and satisfies 1≤i≤n, j is the user number and satisfies 1≤j≤m, and the score value can be a binary attribute value or a numerical score. Usually, the number of projects and users is very large, and the formed matrix is relatively sparse. If the matrix is directly used to generate recommendations, it will not be able to produce good ...
Embodiment 3
[0084] Combined with the following specific formulas, figure 2 and image 3 The scheme in embodiment 1 and 2 is introduced in detail, see the following description for details:
[0085] Two movie rating datasets, Netflix and MovieLens, are used for evaluation. Take the MovieLens data as the target data set and divide it into the training set T R and the test set T E , choose T randomly E5, 10, 20, and 30 evaluations of each user in the set are used as observation sets. In order to avoid the randomness of the experiment, in all experiments, according to the target training score set T R When generating observation scores, the experiment was randomly run 5 times and the average value was taken.
[0086] Two evaluation methods were used: mean absolute error (MAE) and root mean square error (RMSE).
[0087] M A E = Σ ( i , ...
PUM
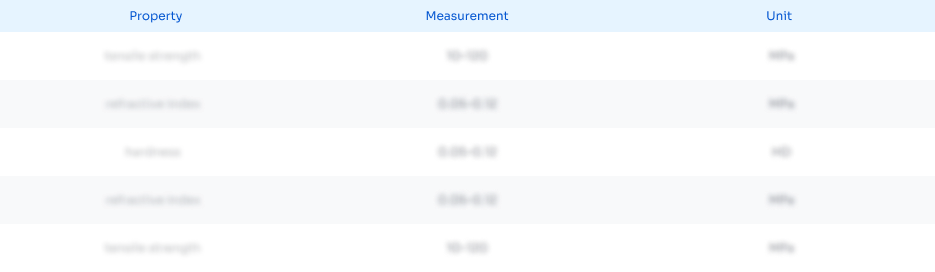
Abstract
Description
Claims
Application Information
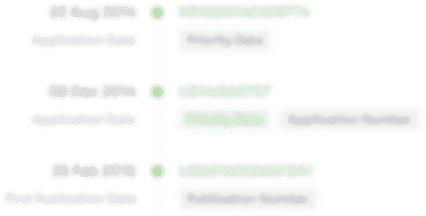
- R&D
- Intellectual Property
- Life Sciences
- Materials
- Tech Scout
- Unparalleled Data Quality
- Higher Quality Content
- 60% Fewer Hallucinations
Browse by: Latest US Patents, China's latest patents, Technical Efficacy Thesaurus, Application Domain, Technology Topic, Popular Technical Reports.
© 2025 PatSnap. All rights reserved.Legal|Privacy policy|Modern Slavery Act Transparency Statement|Sitemap|About US| Contact US: help@patsnap.com