Large-scale traffic network jam prediction method and device based on convolutional neural network
A convolutional neural network and traffic network technology, which is applied in the field of large-scale traffic network congestion prediction, can solve the problems of not being able to understand the spatial relationship of data, not being able to consider the relationship of traffic network at the same time, and not being able to handle multi-output problems well. The effect of improving model calculation efficiency, reducing urban carbon dioxide emissions, and improving road operation efficiency
- Summary
- Abstract
- Description
- Claims
- Application Information
AI Technical Summary
Problems solved by technology
Method used
Image
Examples
Embodiment Construction
[0026] In order to make the purpose, technical solutions and advantages of the embodiments of the present invention clearer, the technical solutions in the embodiments of the present invention will be clearly described below in conjunction with the accompanying drawings in the embodiments of the present invention. Obviously, the described embodiments are the Some, but not all, embodiments are invented. Based on the embodiments of the present invention, all other embodiments obtained by persons of ordinary skill in the art without making creative efforts belong to the protection scope of the present invention.
[0027] see figure 1 , this embodiment discloses a large-scale traffic network congestion prediction method based on a convolutional neural network, including:
[0028] S1. Collect the GPS data of the vehicle, and extract the vehicle operation data of each road section at each moment, and generate a matrix M according to the obtained vehicle operation data, wherein the ...
PUM
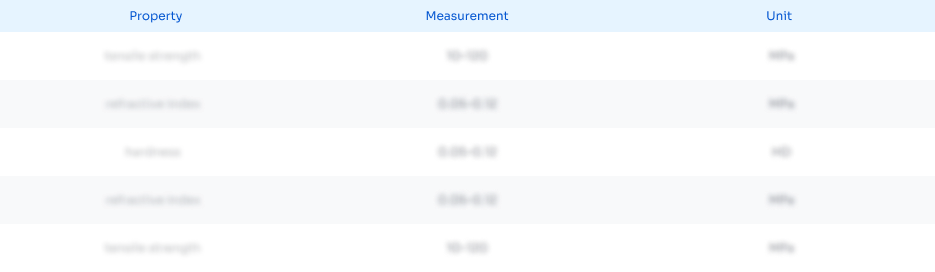
Abstract
Description
Claims
Application Information
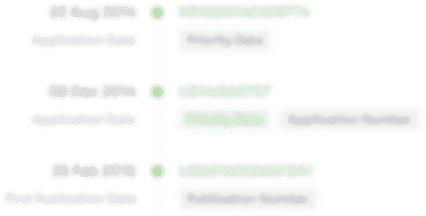
- R&D
- Intellectual Property
- Life Sciences
- Materials
- Tech Scout
- Unparalleled Data Quality
- Higher Quality Content
- 60% Fewer Hallucinations
Browse by: Latest US Patents, China's latest patents, Technical Efficacy Thesaurus, Application Domain, Technology Topic, Popular Technical Reports.
© 2025 PatSnap. All rights reserved.Legal|Privacy policy|Modern Slavery Act Transparency Statement|Sitemap|About US| Contact US: help@patsnap.com