Device and method for forecasting survival rate of freshwater fish based on passive acoustic information
A technology for predicting device and survival rate, applied in fish farming, radio wave measurement system, instrument, etc., can solve undiscovered problems, achieve the effects of avoiding adverse effects, non-destructive testing, and reducing farming or transportation costs
- Summary
- Abstract
- Description
- Claims
- Application Information
AI Technical Summary
Problems solved by technology
Method used
Image
Examples
Embodiment 1
[0045] A freshwater fish survival rate prediction device based on passive acoustic information, the device for extracting the original fish sound signal includes a first fish box 1, a second fish box 2, and a first hydrophone 4 is arranged in the first fish box 1 , the second fish tank 2 is provided with a second hydrophone 5, and the first hydrophone 4 and the second hydrophone 5 are connected to the acoustic recorder 3 respectively. The first fish box is used to collect freshwater fish sound signals, and the second fish box is used to collect background environmental noise, which is used for background environmental noise denoising during post-data processing.
[0046] The first fish tank 1, the first hydrophone 4, and the second hydrophone 5 are provided with several. When used for transportation or farming, there are multiple first fish boxes for placing fish, so each fish box for placing fish needs to collect fish sound signals.
[0047] In the process of large-scale bre...
Embodiment 2
[0050] Example 2 Crucian carp survival rate prediction model
[0051] 1) Acquisition and denoising processing of fish sound signal: respectively in figure 1 Inject 500L of water into the first fish box and the second fish box, the water temperature is 10-15°C, the dissolved oxygen is 7-8mg / L, and the pH is 7.2-7.5, and the first hydrophone and the second water The listener is placed 20cm below the water surface. Put the crucian carp into the No. 1 fish box. The number of live crucian carp is 1-50, the minimum fish-to-water ratio is 1:999, and the maximum fish-to-water ratio is 1:19. Let it stand for 5 minutes until the fish is relatively stable in the water , set the acoustic recorder to collect fish sound signals.
[0052] The setting parameters are as follows: acquisition time: 1min; sampling frequency: 4000Hz; acquisition channel: dual channel; acquisition frequency: 3 times. A total of 1363 crucian carp sound signal samples were collected.
[0053] 2) Extract character...
Embodiment 3
[0085] Example 3 Bream Survival Rate Prediction Model
[0086] 1) Acquisition and denoising processing of fish sound signal: respectively in figure 1 Inject 500L of water into the first fish box and the second fish box, the water temperature is 10-15°C, the dissolved oxygen is 7-8mg / L, and the pH is 7.2-7.5, and the first hydrophone and the second water The listener is placed 20cm below the water surface. Put the crucian carp into the No. 1 fish box. The number of live fish of bream ranges from 1 to 30. The minimum fish-to-water ratio is 1:666, and the maximum fish-to-water ratio is 1:21. Let it stand for 5 minutes until the fish is relatively stable in the water , set the acoustic recorder to collect fish sound signals.
[0087] The setting parameters are as follows: acquisition time: 1min; sampling frequency: 4000Hz; acquisition channel: dual channel; acquisition frequency: 3 times. There are 294 samples of bream sound signal.
[0088] 2) extract characteristic parameter...
PUM
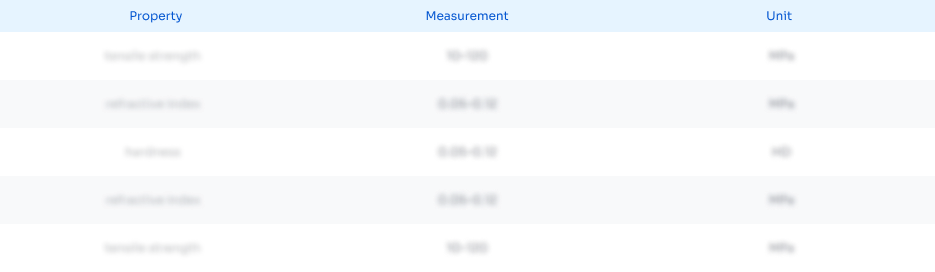
Abstract
Description
Claims
Application Information
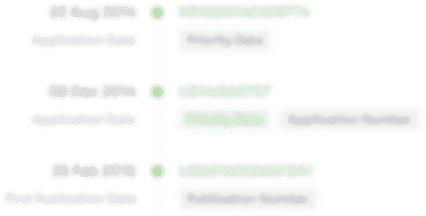
- Generate Ideas
- Intellectual Property
- Life Sciences
- Materials
- Tech Scout
- Unparalleled Data Quality
- Higher Quality Content
- 60% Fewer Hallucinations
Browse by: Latest US Patents, China's latest patents, Technical Efficacy Thesaurus, Application Domain, Technology Topic, Popular Technical Reports.
© 2025 PatSnap. All rights reserved.Legal|Privacy policy|Modern Slavery Act Transparency Statement|Sitemap|About US| Contact US: help@patsnap.com