Accelerating degradation model for photovoltaic component constructed on basis of deep learning method and forecasting method for service life of photovoltaic component
A photovoltaic module and accelerated degradation technology, applied in the direction of neural learning methods, biological neural network models, informatics, etc., can solve the problems of many stress factors and difficulties in the performance degradation of photovoltaic modules, and the difficulty of clarifying the functional relationship
- Summary
- Abstract
- Description
- Claims
- Application Information
AI Technical Summary
Problems solved by technology
Method used
Image
Examples
Embodiment 1
[0041] The accelerated degradation model of photovoltaic modules based on the deep learning method and the life prediction method of photovoltaic modules provided in this embodiment include the following steps:
[0042] (1) Acquisition of initial data: Select photovoltaic modules, set and establish accelerated degradation model with different accelerated stress level combinations S i(i=1,2,3….n, representing different accelerated stress combinations), S i including temperature T i , Humidity H i and light radiation Ra i , conduct accelerated degradation experiments to obtain the output power P of photovoltaic modules under accelerated degradation conditions di , according to the output power P di Obtain the pseudo-failure life, and obtain the pseudo-failure life distribution quantile function Q according to the pseudo-failure life i (p), the different accelerated stress conditions S i , Pseudo-failure life distribution quantile function Q i (p) as initial data;
[0043...
Embodiment 2
[0059] The accelerated degradation model of photovoltaic modules based on the deep learning method and the life prediction method of photovoltaic modules provided in this embodiment include the following steps:
[0060] (1) Acquisition of initial data: Select photovoltaic modules, set and establish accelerated degradation model with different accelerated stress level combinations S i , S i including temperature T i , Humidity H i and light radiation Ra i , conduct accelerated degradation experiments, obtain the output power of photovoltaic modules under accelerated degradation conditions, and obtain the pseudo-failure life distribution quantile function Q according to the output power i (p), the different accelerated stress conditions S i , Pseudo-failure life distribution quantile function Q i (p) as initial data;
[0061] The specific process is as follows:
[0062] 1.1. Accelerated degradation test
[0063] The accelerated degradation test system used to obtain perf...
PUM
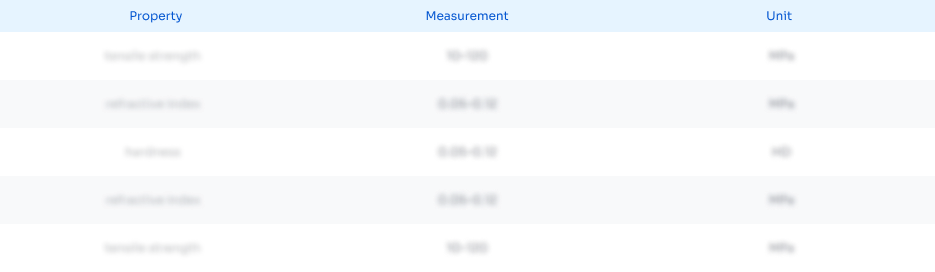
Abstract
Description
Claims
Application Information
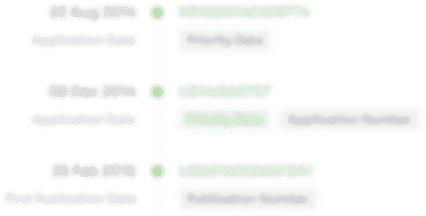
- R&D Engineer
- R&D Manager
- IP Professional
- Industry Leading Data Capabilities
- Powerful AI technology
- Patent DNA Extraction
Browse by: Latest US Patents, China's latest patents, Technical Efficacy Thesaurus, Application Domain, Technology Topic, Popular Technical Reports.
© 2024 PatSnap. All rights reserved.Legal|Privacy policy|Modern Slavery Act Transparency Statement|Sitemap|About US| Contact US: help@patsnap.com