Machine learning method for identifying origin of Wuyi rock tea automatically
A technology of machine learning and origin, applied in instruments, scientific instruments, material analysis through optical means, etc., can solve the problem that the detection data cannot represent the key information of origin traceability
- Summary
- Abstract
- Description
- Claims
- Application Information
AI Technical Summary
Problems solved by technology
Method used
Image
Examples
Embodiment 1
[0080] A. Collect rock tea samples from different origins
[0081] The national standard (GB / T 18745-2006) stipulates the scope of geographical protection of Wuyi rock tea, that is, within the administrative division of Wuyishan City, Fujian Province, the present invention is located in Wuyi Street, Chong'an Street, Shangmei, and Xingxia in the Wuyi Rock Tea Geographical Indication Protection Area. Samples were collected in 11 administrative areas including Village, Wufu, Langu, Xinfeng Street, Yangzhuang, Xingtian, Xiamei, and Wutun, and 3 sampling points were randomly selected in each administrative area (respectively A, B, C to be marked), a total of 33 sampling points, the sampling range basically covers the main production areas, and each sampling point takes 15 samples (respectively marked with A-1, A-2...A-15), and obtained 495 samples of Wuyi rock tea in geographical indication protected areas, and other counties and cities in Fujian Province except Wuyishan City (Jian...
Embodiment 2
[0153] Adopt the modeling method identical with embodiment 1, data segmentation uses Kenstone segmentation procedure, with Monte Carlo interactive verification, set up neural network ELM, partial least squares (PLSDA), least squares support vector machine (LS-SVM) respectively In the model, the near-infrared data remains unchanged, and the stable isotopes, trace elements, amino acids, and electronic tongues are respectively classified according to hydrogen, oxygen, nitrogen, carbon, strontium, Cs, Cu, Ca, Rb, Sr, Ba, asparagine, proline, The model recognition rates of tryptophan, phosphoethanolamine, urea, valine, ZZ, BA, BB, CA, GA, HA, and JB spliced in near-infrared data were 90.5%, 86.7%, and 78.9%, respectively.
Embodiment 3
[0155] Using the same modeling method as in Example 1, the data segmentation uses the Kenstone segmentation program, and uses the Monte Carlo interactive verification to establish the neural network ELM, PLSDA, and LS-SVM discriminant models respectively. The near-infrared data is unchanged, and stable isotopes and trace elements , amino acid, electronic tongue data according to hydrogen, oxygen, nitrogen, carbon, strontium, Cs, Cu, Ca, Rb, Sr, Ba, asparagine, proline, tryptophan, phosphoethanolamine, ZZ, BA, BB, After splicing CA, GA, HA, and JB into near-infrared data, the model recognition rates are 91.2%, 87.9%, and 82.3%, respectively.
PUM
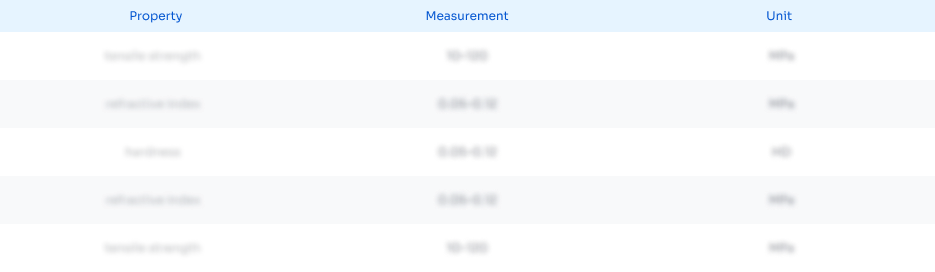
Abstract
Description
Claims
Application Information
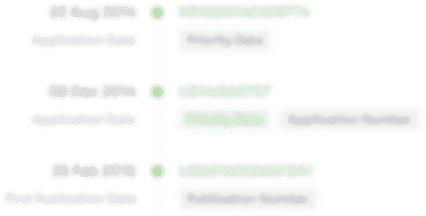
- R&D
- Intellectual Property
- Life Sciences
- Materials
- Tech Scout
- Unparalleled Data Quality
- Higher Quality Content
- 60% Fewer Hallucinations
Browse by: Latest US Patents, China's latest patents, Technical Efficacy Thesaurus, Application Domain, Technology Topic, Popular Technical Reports.
© 2025 PatSnap. All rights reserved.Legal|Privacy policy|Modern Slavery Act Transparency Statement|Sitemap|About US| Contact US: help@patsnap.com