Combined medical science image fusion denoising method based on discrimination dictionary learning
A medical image and dictionary learning technology, which is applied in the field of joint medical image fusion and denoising, can solve the problems of joint medical image denoising and fusion difficulties, achieve clear edge details, improve fusion effect, and avoid the effect of oscillation response propagation
- Summary
- Abstract
- Description
- Claims
- Application Information
AI Technical Summary
Problems solved by technology
Method used
Image
Examples
Embodiment 1
[0033] Embodiment 1: as figure 1 As shown, the present invention proposes a method for joint medical image fusion and denoising based on discriminant dictionary learning. Firstly, the image used for training is collected, and then the collected image is trained to obtain a sparse dictionary and a low-rank dictionary, and then the sparse Image decomposition of dictionaries and low-rank dictionaries to obtain sparse elements and low-rank elements, add sparse constraints to sparse elements, and add kernel norm and weighted kernel norm constraints to low-rank elements to obtain low-rank coefficients and sparse coefficients respectively. The rank dictionary and the low-rank coefficients are iteratively updated to obtain the updated low-rank components, and the sparse dictionary and the sparse coefficients are iteratively updated to obtain the sparse components. Finally, the low-rank components and the sparse components are fused to obtain the final fused image.
[0034] Step1, firs...
Embodiment 2
[0082] Embodiment 2: 8 images are collected in the process of training the dictionary. The initial low-rank dictionary and sparse dictionary are obtained by passing the training sample image through K-SVD. According to the proposed dictionary learning algorithm iteratively updated to obtain the required sparse dictionary D s and a low-rank dictionary D l ; In the image fusion method when the input source image has no noise, the figure 2 The source image of the source image is separated by the existing RPCA algorithm to obtain the low-rank component and sparse component of the source image; the parameters involved in the fusion algorithm η1, η2, η3, η4, η5 are set to 1, 1, 1, 0, 1, The number of iterations in dictionary learning is 5. Through the calculation of the image fusion algorithm, the fusion result obtained in the implementation of the matlab software is Figure 4 The last image in Table 1 shows the evaluation index of noise-free source image fusion and traditional...
Embodiment 3
[0086] Embodiment 3: The method of dictionary training is the same as example 1. In the image fusion method, when the input source image is destroyed by Gaussian white noise, the image 3 The source image, η1, η2, η3, η4, η5 are set to 1, 1, 1, 1.3, 1 respectively. The number of iterations is 10. Other operations are the same as Example 1. The noise source image used is destroyed by Gaussian white noise with a noise level of 10 or 20, and the obtained fusion result is Figure 5 The last image in . Table 2 shows the evaluation indicators of Gaussian white noise source image denoising fusion and traditional methods, and the maximum value of each set of evaluation data is enlarged and bolded.
[0087]
[0088] Table 2: The average quantitative evaluation of 10 groups of medical image noise by different methods
PUM
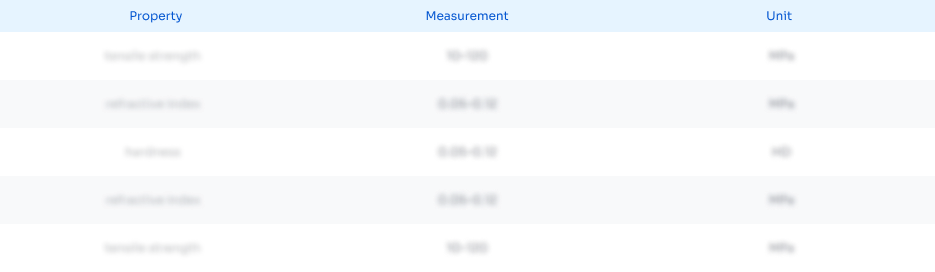
Abstract
Description
Claims
Application Information
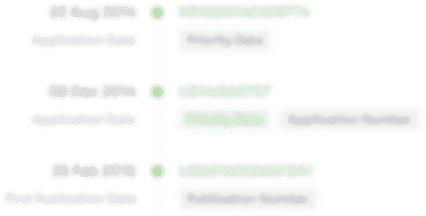
- R&D
- Intellectual Property
- Life Sciences
- Materials
- Tech Scout
- Unparalleled Data Quality
- Higher Quality Content
- 60% Fewer Hallucinations
Browse by: Latest US Patents, China's latest patents, Technical Efficacy Thesaurus, Application Domain, Technology Topic, Popular Technical Reports.
© 2025 PatSnap. All rights reserved.Legal|Privacy policy|Modern Slavery Act Transparency Statement|Sitemap|About US| Contact US: help@patsnap.com