Method for predicting human body thoracico-abdominal surface area respiratory movement in radiotherapy
A technology of surface area and breathing movement, applied in radiation therapy, X-ray/γ-ray/particle irradiation therapy, treatment, etc., can solve the problems of normal tissue damage, poor patient tolerance, low treatment efficiency, etc., to improve curative effect, Increased applicability and easy parameter optimization
- Summary
- Abstract
- Description
- Claims
- Application Information
AI Technical Summary
Problems solved by technology
Method used
Image
Examples
specific Embodiment 1
[0025] A method for predicting respiratory movement of the human chest and abdomen surface area during radiotherapy in this embodiment includes the following steps:
[0026] Step a. Use the light pattern projector to project a color pattern composed of three different frequency RGB cosine curves onto the surface of the chest and abdomen of the human body. A 3CCD color camera is placed on both sides of the light pattern projector to collect the scene image and send it to the computer. Perform post-processing, using dual cameras to obtain the three-dimensional coordinates of feature marker points and area boundary lines based on the principle of binocular vision;
[0027] Step b. For the two video sequences acquired by the left and right cameras, after extracting the same area and its boundary lines and feature marker points in the corresponding image pair, match the area boundary lines and feature marker points respectively, and then use the left and right cameras to The princi...
PUM
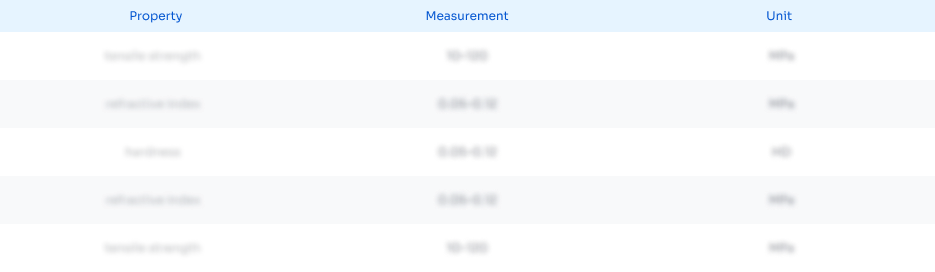
Abstract
Description
Claims
Application Information
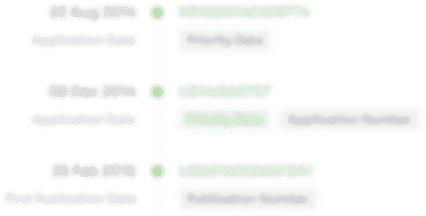
- Generate Ideas
- Intellectual Property
- Life Sciences
- Materials
- Tech Scout
- Unparalleled Data Quality
- Higher Quality Content
- 60% Fewer Hallucinations
Browse by: Latest US Patents, China's latest patents, Technical Efficacy Thesaurus, Application Domain, Technology Topic, Popular Technical Reports.
© 2025 PatSnap. All rights reserved.Legal|Privacy policy|Modern Slavery Act Transparency Statement|Sitemap|About US| Contact US: help@patsnap.com