Nuclear extreme learning machine quantile regression-based wind power interval prediction method
A kernel extreme learning machine, quantile regression technology, applied in prediction, machine learning, biological model and other directions, can solve the problems of complex calculation, difficult parameters, low reliability, etc., to achieve strong fitting ability, less parameters, model simple effect
- Summary
- Abstract
- Description
- Claims
- Application Information
AI Technical Summary
Problems solved by technology
Method used
Image
Examples
Embodiment Construction
[0034] The embodiments will be described in detail below in conjunction with the accompanying drawings.
[0035] Such as figure 1 As shown, it is a schematic flow chart of a wind power interval prediction method based on kernel extreme learning machine quantile regression of the present invention. The embodiment of the present invention uses the actual wind power data collected from a certain wind farm in Northwest China from the field, with a resolution of 15 minutes, including the measured output power and the wind speed of the anemometer tower, and performs interval prediction of its power. The method includes the following steps:
[0036] Step 1. Collect the original data of the wind farm to form the original data set D={(w 1 ,p1 )(w 2 ,p 2 )…(w i ,p i )}, w i is the wind speed at the i-th moment, p i is the power at the i-th moment, and perform data processing: .
[0037] The raw data processing of the wind farm is sorted in chronological order, and the missing p...
PUM
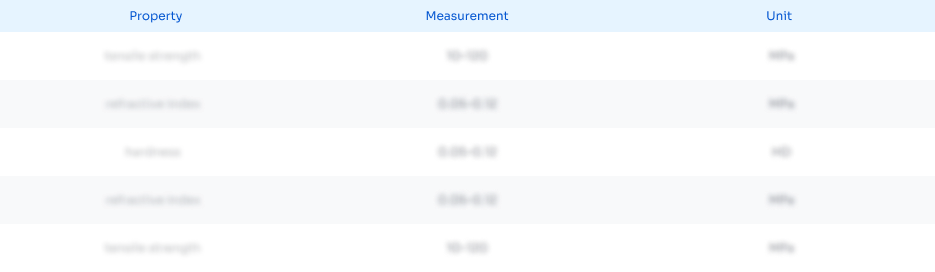
Abstract
Description
Claims
Application Information
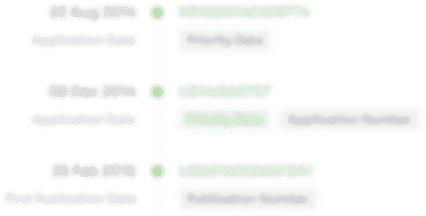
- R&D Engineer
- R&D Manager
- IP Professional
- Industry Leading Data Capabilities
- Powerful AI technology
- Patent DNA Extraction
Browse by: Latest US Patents, China's latest patents, Technical Efficacy Thesaurus, Application Domain, Technology Topic, Popular Technical Reports.
© 2024 PatSnap. All rights reserved.Legal|Privacy policy|Modern Slavery Act Transparency Statement|Sitemap|About US| Contact US: help@patsnap.com