Frequency domain convolution blind source separation multi-band and multi-centroid clustering sorting method
A technology of blind source separation and frequency domain convolution, applied in speech analysis, instruments, etc., can solve problems such as difficulty in finding sorting, consistent global reference, and poor correlation, and achieve improved clustering efficiency and reliable position alignment performance. , the effect of improving the accuracy
- Summary
- Abstract
- Description
- Claims
- Application Information
AI Technical Summary
Problems solved by technology
Method used
Image
Examples
Embodiment Construction
[0062] The present invention is further described below in conjunction with accompanying drawing:
[0063] see Figure 1-Figure 12 , the frequency-domain convolutional blind source separation sub-band multi-centroid clustering sorting method is characterized in that, using the convolutional mixture model, for the convolutional mixed observation signal, firstly use the short-time Fourier transform to convert the signal from the time domain to In the time-frequency domain, the complex signal is blindly separated using the complex ICA algorithm on each frequency band; then sorted by the following steps:
[0064] Step 1, overlapping and grouping the full-band signals: divide the entire frequency band into several sub-bands, and there are overlapping frequency bands between the sub-bands;
[0065] Step 2, clustering and sorting in sub-bands: using the high correlation of the amplitude envelopes of adjacent frequency segments of the same signal in the frequency domain, and the unco...
PUM
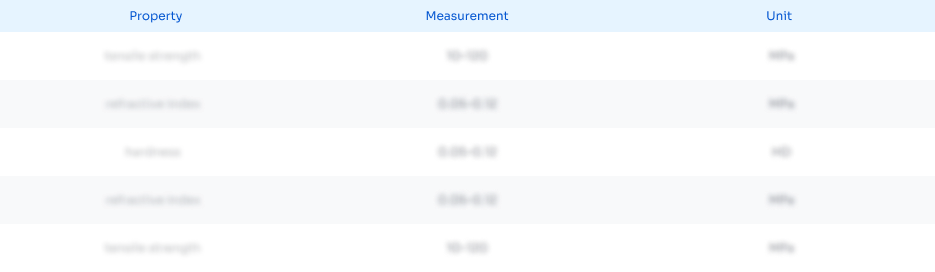
Abstract
Description
Claims
Application Information
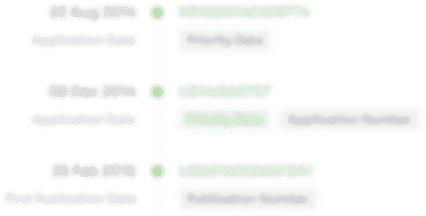
- R&D Engineer
- R&D Manager
- IP Professional
- Industry Leading Data Capabilities
- Powerful AI technology
- Patent DNA Extraction
Browse by: Latest US Patents, China's latest patents, Technical Efficacy Thesaurus, Application Domain, Technology Topic, Popular Technical Reports.
© 2024 PatSnap. All rights reserved.Legal|Privacy policy|Modern Slavery Act Transparency Statement|Sitemap|About US| Contact US: help@patsnap.com