Blurred variable image blind restoration method based on deep convolution network
A deep convolution and blurred image technology, applied in image enhancement, image analysis, image data processing and other directions, can solve the problems of lack of universality, slow running speed, and block accuracy affecting the restoration effect.
- Summary
- Abstract
- Description
- Claims
- Application Information
AI Technical Summary
Problems solved by technology
Method used
Image
Examples
Embodiment
[0062] This embodiment provides a method for blind restoration of fuzzy and variable images based on deep convolutional networks. The flow chart of the method is as follows Figure 4 shown, including the following steps:
[0063] S1. Model the blur types that need to be considered in the blurred image, and determine the range of blur parameters for each blur type; wherein, the blur model obtained by modeling the blur types that need to be considered in the blurred image is defined according to the following formula:
[0064] I b,ij =[q(x|i,j)*I o +ε]
[0065] where I b Represents the observed blurred image, I o represents the underlying sharp image, I b,ij and I o,ij represent the values of the blurred image and the clear image at the position [i,j] (i row j column) respectively, Indicates the operation of taking the position of [i, j], q(·|i, j) represents a variable PSF (point spread function, point spread function), ε is additive noise, x=(x 1 ,x 2 ) represent...
PUM
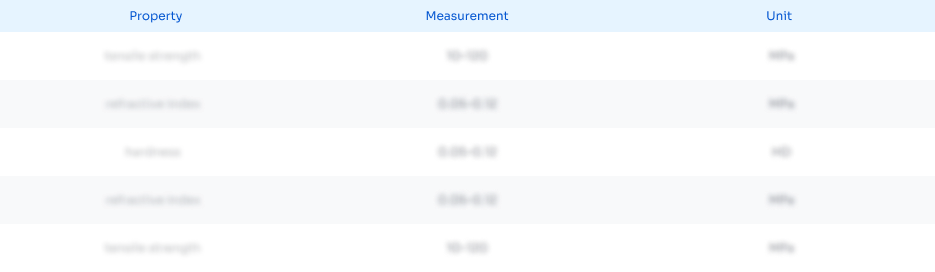
Abstract
Description
Claims
Application Information
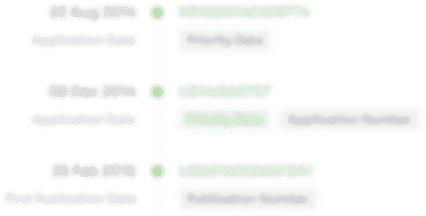
- R&D
- Intellectual Property
- Life Sciences
- Materials
- Tech Scout
- Unparalleled Data Quality
- Higher Quality Content
- 60% Fewer Hallucinations
Browse by: Latest US Patents, China's latest patents, Technical Efficacy Thesaurus, Application Domain, Technology Topic, Popular Technical Reports.
© 2025 PatSnap. All rights reserved.Legal|Privacy policy|Modern Slavery Act Transparency Statement|Sitemap|About US| Contact US: help@patsnap.com