Thyroid tumor pathological tissue section image classification method and device
A technology for thyroid tumors and tissue slicing, applied in image analysis, image enhancement, image data processing, etc., can solve problems such as time-consuming and labor-intensive, and not clinically practical
- Summary
- Abstract
- Description
- Claims
- Application Information
AI Technical Summary
Problems solved by technology
Method used
Image
Examples
Embodiment 1
[0068] Example 1 Obtain and classify the original image set of thyroid tumor pathological tissue slices
[0069] The pathological section data set used in the present invention comes from the actual application site. The data set comes from the Fudan University Affiliated Tumor Hospital. It is obtained from pathological section specimens prepared by resection of thyroid tumors. The hospital regards such pathological tissue sections as being It is an important diagnostic basis for diagnosing cancer, so it is of great significance to use this type of data set to build an automated diagnosis system. After we obtain the slice data set, we need to classify it first. There are 7 categories: normal, adenoma, thyroid nodule, papillary carcinoma, follicular carcinoma, medullary carcinoma and undifferentiated carcinoma. Labeled as 0-6, this is an encoding process that converts abstract information into digital information. The normal data set has 93 original pathological slice images, the...
Embodiment 2
[0070] Example 2 Fully automated interception of sub-image set data amplification
[0071] Deep learning training requires a large amount of training data support to achieve satisfactory classification results. In the medical field, due to privacy protection, it is often difficult to collect a large number of data sets to meet the training requirements. Due to the limited number of data sets obtained, there are only 632, but the system needs to classify 7 categories, so we need to expand the data set. The previous amplification method was mainly used by professional doctors to find the denser tumor cells in the pathological section to intercept. According to this method, one pathological section can amplify several pictures containing certain tumor cells. The amplification method is very time-consuming and cumbersome to operate, and professional doctors often do not have enough time. Therefore, this embodiment adopts the fully automatic interception amplification method, and the ...
Embodiment 3
[0086] Example 3 Using flip and / or rotation to perform a second data augmentation on the training set
[0087] The second data augmentation of the training set is based on the horizontal flip and / or rotation of the sub-images. The horizontal flip can expand the data set to twice the original size, and rotate 0 degrees, 90 degrees, 180, and 270 degrees. The original training set is expanded four times, so the original data set can be expanded eight times after horizontal flipping and rotation.
[0088] The second amplification of the training set is to use the online data amplification method. Compared with the traditional pre-amplification method before training, this online amplification method has the advantage of reducing the occupation of the amplified data set. Storage space. It only needs to adopt the method of batch training. During training, each sub-image in each batch of training is randomly flipped and / or rotated. When the number of training iterations is large enough, ...
PUM
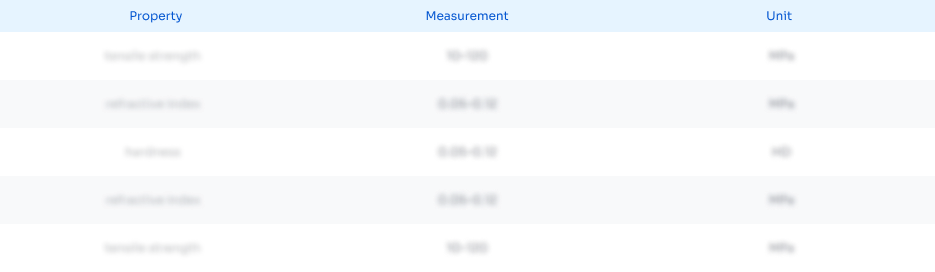
Abstract
Description
Claims
Application Information
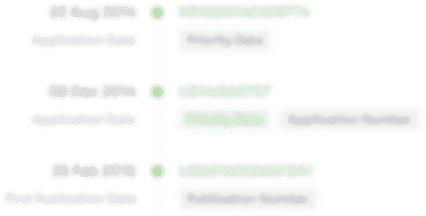
- R&D Engineer
- R&D Manager
- IP Professional
- Industry Leading Data Capabilities
- Powerful AI technology
- Patent DNA Extraction
Browse by: Latest US Patents, China's latest patents, Technical Efficacy Thesaurus, Application Domain, Technology Topic.
© 2024 PatSnap. All rights reserved.Legal|Privacy policy|Modern Slavery Act Transparency Statement|Sitemap