An Image Processing Method Based on Matrix Variable Variational Autoencoder
An image processing and autoencoder technology, applied in the field of computer vision and machine learning, which can solve problems such as destroying the spatial structure
- Summary
- Abstract
- Description
- Claims
- Application Information
AI Technical Summary
Problems solved by technology
Method used
Image
Examples
Embodiment Construction
[0009] The invention provides a method for image processing based on a matrix variable variational autoencoder (Matrix-variate VariationalAutoencoder, MVVAE),
[0010] Suppose there are N independent and identically distributed image sets Each image is represented as That is, the size of the input sample is a two-dimensional matrix of I×J. The present invention aims to model the statistical distribution logp of a set of images θ (X), and then effectively perform image reconstruction, denoising and completion. The image modeling process is based on the MVVAE network proposed by the present invention, so the core is to model the network and train to obtain the parameters of the network model.
[0011] To achieve the above object, the present invention adopts the following technical solutions:
[0012] 1. Definition of MVVAE model for image set distribution modeling
[0013] MVVAE model definition, such as figure 1 as shown,
[0014] in this model Is the input layer ma...
PUM
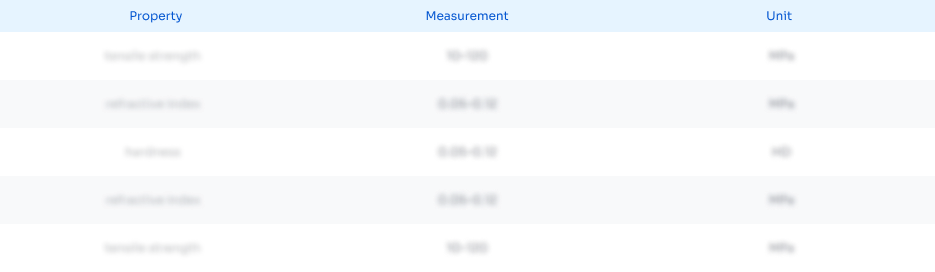
Abstract
Description
Claims
Application Information
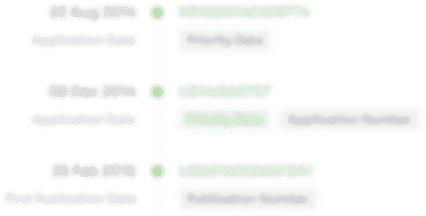
- Generate Ideas
- Intellectual Property
- Life Sciences
- Materials
- Tech Scout
- Unparalleled Data Quality
- Higher Quality Content
- 60% Fewer Hallucinations
Browse by: Latest US Patents, China's latest patents, Technical Efficacy Thesaurus, Application Domain, Technology Topic, Popular Technical Reports.
© 2025 PatSnap. All rights reserved.Legal|Privacy policy|Modern Slavery Act Transparency Statement|Sitemap|About US| Contact US: help@patsnap.com