Seismic wave recognition algorithm based on convolution neural network
A convolutional neural network and natural seismic wave technology, applied in the field of deep learning, can solve the problems of high data volume and accuracy requirements, upper limit of recognition accuracy, and insufficient accuracy.
- Summary
- Abstract
- Description
- Claims
- Application Information
AI Technical Summary
Problems solved by technology
Method used
Image
Examples
Embodiment Construction
[0026] The following is attached with the manual Figure 1-5 The present invention is further described in detail.
[0027] A seismic wave recognition algorithm based on convolutional neural network, step 1: model training data acquisition;
[0028] This method first uses the STA / LTA method to find the position of the p-wave starting point in the seismic data, and intercepts 169 seconds from the p-wave starting point, that is, 16,900 data points as training data for a single seismic event at a single station in this dimension. Any seismic event is recorded by three latitudes: north-south, east-west, and perpendicular to the surface. Therefore, for a single earthquake event at a single station, the model will obtain a vector with a size of 3×16900. In the process of adding the vector to the training set, the sliding window method of averaging is used to control the data scale, and the data on the three latitudes are spliced in the order of east-west, north-south, and vertica...
PUM
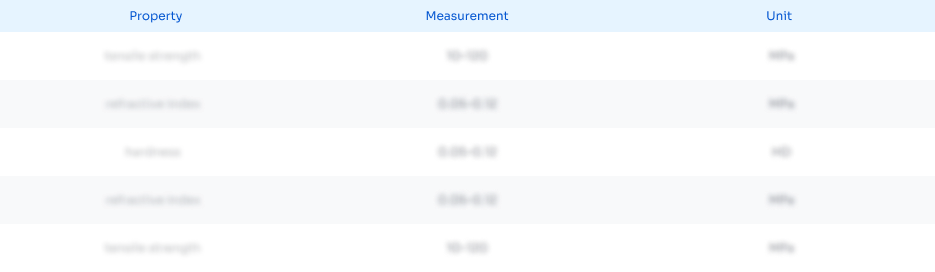
Abstract
Description
Claims
Application Information
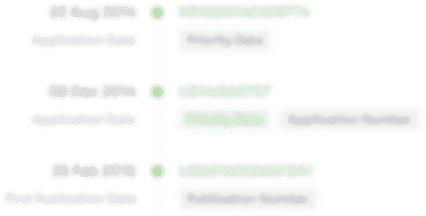
- R&D Engineer
- R&D Manager
- IP Professional
- Industry Leading Data Capabilities
- Powerful AI technology
- Patent DNA Extraction
Browse by: Latest US Patents, China's latest patents, Technical Efficacy Thesaurus, Application Domain, Technology Topic, Popular Technical Reports.
© 2024 PatSnap. All rights reserved.Legal|Privacy policy|Modern Slavery Act Transparency Statement|Sitemap|About US| Contact US: help@patsnap.com