PMU primary frequency modulation load forecasting method based on LSTM and associative full-connected neural network
A neural network and load forecasting technology, applied in neural learning methods, biological neural network models, instruments, etc., can solve problems such as difficulty in wavelet transform algorithm, difficulty in processing large-scale training samples, and rough analysis by support vector machines, and achieve stable operation. Solid guarantee, solving long-term dependency problems, and the effect of short running time
- Summary
- Abstract
- Description
- Claims
- Application Information
AI Technical Summary
Problems solved by technology
Method used
Image
Examples
Embodiment
[0060] The present invention provides a PMU primary frequency modulation load prediction method based on an associated fully connected neural network and LSTM, the steps of which are as follows:
[0061] The historical data of the target to be predicted is used as the original data, after data longitudinal comparison processing and normalization, 80% of the processed data (x, y) is selected as the training data (x_train, y_train), and the remaining 20% (x_valid ,y_valid) is used to verify the accuracy of the prediction method, set: the batch size batch_size is 64 and the window size window is 30;
[0062] The data longitudinal comparison processing method is as follows:
[0063] Compare the load value at time t with the data of the load value at the previous time, if there is no change, then judge that the data is dead zone data and remove it;
[0064] Data normalization adopts the most value method, and the formula is as follows:
[0065]
[0066] In the formula, L ma...
PUM
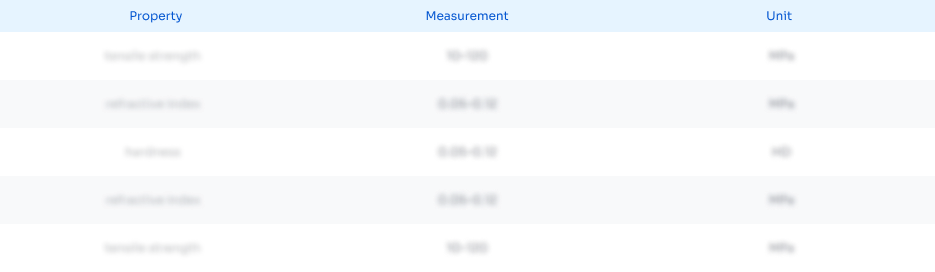
Abstract
Description
Claims
Application Information
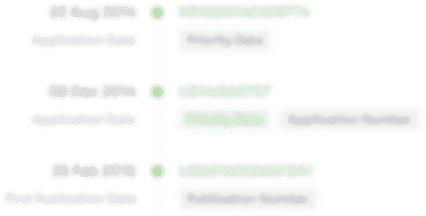
- R&D Engineer
- R&D Manager
- IP Professional
- Industry Leading Data Capabilities
- Powerful AI technology
- Patent DNA Extraction
Browse by: Latest US Patents, China's latest patents, Technical Efficacy Thesaurus, Application Domain, Technology Topic.
© 2024 PatSnap. All rights reserved.Legal|Privacy policy|Modern Slavery Act Transparency Statement|Sitemap