SAR image iron tower target detection method based on deep learning
A target detection and deep learning technology, applied in the field of image processing, can solve problems such as poor detection performance
- Summary
- Abstract
- Description
- Claims
- Application Information
AI Technical Summary
Problems solved by technology
Method used
Image
Examples
Embodiment 1
[0078] Such as figure 1 As shown, an embodiment of the present invention provides a deep learning-based SAR image iron tower target detection method, including:
[0079] S1. Randomly extract multiple SAR images from the SAR data set, segment to obtain sample slices, set a sample label for each sample slice, and then construct a training sample set. The sample label includes the coordinate information and category information of the target in the sample slice.
[0080] Preferably, step S1 includes:
[0081] S1-1. Randomly extract 100 SAR images from the MiniSAR data set;
[0082] S1-2. Randomly segment each SAR image to obtain multiple sample slices;
[0083] S1-3. Add a sample label to each sample slice. The sample label includes the absolute path of the sample slice, the number of targets, the coordinates of the target frame (ie coordinate information) and the target category (ie category information); specifically, the sample label The composition can be:
[0084] n x t1 y t1 x b1 y ...
Embodiment 2
[0107] Such as figure 2 As shown, the second embodiment is basically the same as the first embodiment, and the similarities will not be repeated here. The differences are:
[0108] The input image size of the SSD model constructed in step S3 is 300×300 pixels.
[0109] figure 2 It is a schematic diagram of the SSD model in this embodiment, and the key convolutional layer is marked in the figure. Among them, the convolutional layer 4_3 represents the third convolutional layer in the fourth group of convolutional layers, that is, the 10th convolutional layer, the same below.
[0110] Such as figure 2 As shown, the specific structure of the 15-layer convolutional neural network constructed in step S3 includes:
[0111] The first group of convolutional layers: the first 1-2 convolutional layers, each layer uses 64 convolution kernels with a window size of 3×3, the window moving step is 1, the edge is filled with 1 pixel, and the output is 64 sizes 300×300 feature map;
[0112] The seco...
Embodiment 3
[0159] Such as image 3 , Figure 4a with Figure 4b As shown, the third embodiment is basically the same as the second embodiment, and the similarities will not be repeated here. The differences are:
[0160] The data to be tested used in this embodiment is a scene SAR image, such as image 3 Shown. The image size of the data map to be tested is 16384×8192 pixels, which contains a variety of artificial targets such as iron towers and buildings. The purpose is to detect and locate all types of iron tower targets in the data map to be tested.
[0161] In order to better observe the test results, such as Figure 4a with 4b As shown, the part of the target detection result is enlarged, and the part marked with the white frame in the figure is the part of the iron tower detected.
[0162] In this embodiment, by calculating the detection results of the entire data graph to be tested, it is concluded that the accuracy rate of the method provided by the present invention is 82.4%, the recal...
PUM
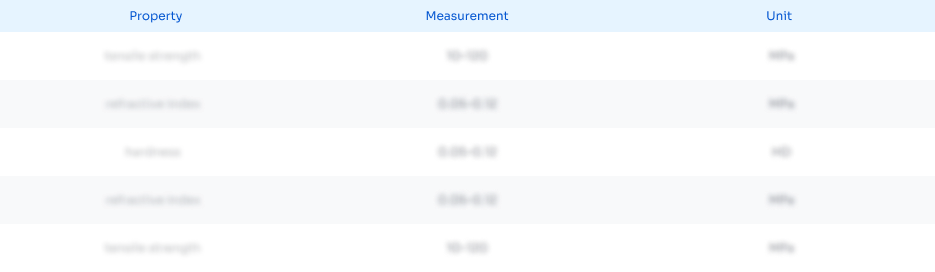
Abstract
Description
Claims
Application Information
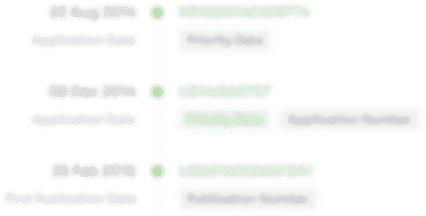
- R&D Engineer
- R&D Manager
- IP Professional
- Industry Leading Data Capabilities
- Powerful AI technology
- Patent DNA Extraction
Browse by: Latest US Patents, China's latest patents, Technical Efficacy Thesaurus, Application Domain, Technology Topic, Popular Technical Reports.
© 2024 PatSnap. All rights reserved.Legal|Privacy policy|Modern Slavery Act Transparency Statement|Sitemap|About US| Contact US: help@patsnap.com