An adaptive enhancement method of brain magnetic resonance volumetric data based on transformed domain HMT model
An adaptive enhancement and magnetic resonance technology, applied in the field of medical image processing, can solve problems such as inability to effectively suppress image noise, easy loss of useful image information, and sensitive threshold selection
- Summary
- Abstract
- Description
- Claims
- Application Information
AI Technical Summary
Problems solved by technology
Method used
Image
Examples
Embodiment Construction
[0098] The present invention proposes a brain magnetic resonance body data adaptive enhancement method of a transform domain HMT model, and the technical scheme flow chart of the method is as follows figure 1 shown. The specific implementation is described as follows.
[0099] The first step is to collect brain MRI data. The resolution of the collected 3T brain magnetic resonance images is 0.6×0.6×0.6mm 3 . Since the whole brain magnetic resonance image is relatively large, it is not easy to analyze the performance of the enhancement method, so a part of the whole brain magnetic resonance image is intercepted, and its size is 64×64×64.
[0100] The second step is three-dimensional discrete wavelet transform. For brain MRI data, the three-dimensional discrete wavelet transform is realized by discrete wavelet transform along the directions of the three coordinate axes respectively. The number of layers of discrete wavelet transform decomposition is 2 to 5 layers.
[0101] ...
PUM
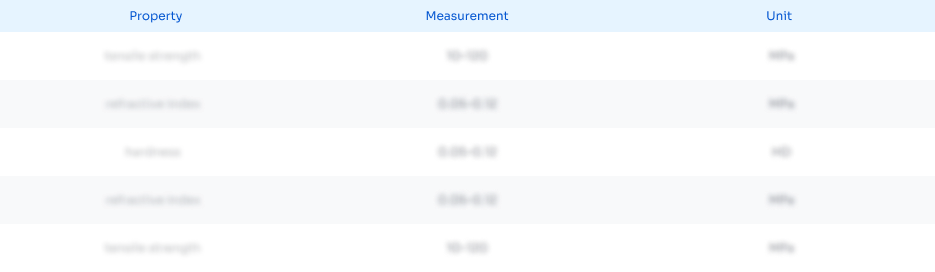
Abstract
Description
Claims
Application Information
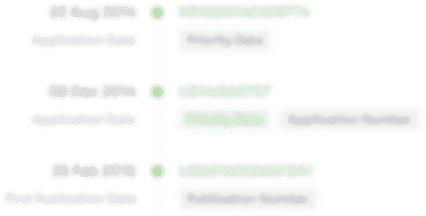
- R&D Engineer
- R&D Manager
- IP Professional
- Industry Leading Data Capabilities
- Powerful AI technology
- Patent DNA Extraction
Browse by: Latest US Patents, China's latest patents, Technical Efficacy Thesaurus, Application Domain, Technology Topic, Popular Technical Reports.
© 2024 PatSnap. All rights reserved.Legal|Privacy policy|Modern Slavery Act Transparency Statement|Sitemap|About US| Contact US: help@patsnap.com