An esophageal cancer pathological image processing method based on depth learning
A pathological image, deep learning technology, applied in the direction of image data processing, image enhancement, image analysis, etc., can solve the problem of no progress, and achieve the effect of being suitable for application and promotion, with significant beneficial effects
- Summary
- Abstract
- Description
- Claims
- Application Information
AI Technical Summary
Problems solved by technology
Method used
Image
Examples
Embodiment Construction
[0033] The present invention will be further described below in conjunction with the accompanying drawings and embodiments.
[0034] Such as figure 1 As shown, a schematic flow chart of the method for processing esophageal cancer pathological images of the present invention is given. If the method for processing pathological images of esophageal cancer is described in a modular form, it consists of an image preprocessing module, a CNN convolutional neural network module, LSTM long-short-term memory network module and classifier module. Image preprocessing performs segmentation and dyeing standardization on the input pathological image, and labels each input image as the input of the CNN convolutional neural network module; The CNN convolutional neural network module first slices each input image vertically, divides each input image into 5 image blocks of the same size, and performs feature extraction on these 5 image blocks, that is, through the CNN convolutional neural networ...
PUM
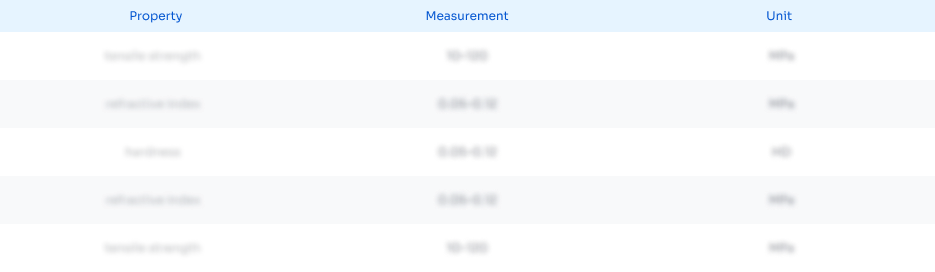
Abstract
Description
Claims
Application Information
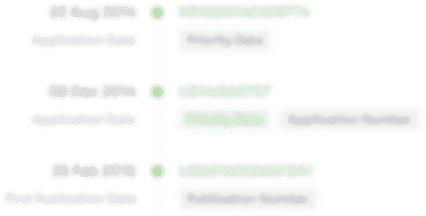
- R&D
- Intellectual Property
- Life Sciences
- Materials
- Tech Scout
- Unparalleled Data Quality
- Higher Quality Content
- 60% Fewer Hallucinations
Browse by: Latest US Patents, China's latest patents, Technical Efficacy Thesaurus, Application Domain, Technology Topic, Popular Technical Reports.
© 2025 PatSnap. All rights reserved.Legal|Privacy policy|Modern Slavery Act Transparency Statement|Sitemap|About US| Contact US: help@patsnap.com