Dynamic neural network adaptive inverse SRM torque control method and system
A technology of dynamic neural network and self-adaptive inverse, applied in the direction of control system, direct torque control, AC motor control, etc., can solve the problem of increasing SRM torque ripple
- Summary
- Abstract
- Description
- Claims
- Application Information
AI Technical Summary
Problems solved by technology
Method used
Image
Examples
Embodiment Construction
[0058] Embodiment of SRM torque control method based on dynamic neural network self-adaptive inversion
[0059] The main steps of the SRM torque control method embodiment of the dynamic neural network self-adaptive inversion are as follows: figure 1 As shown, the details are as follows:
[0060] Ⅰ. PD control
[0061] PD control is proportional and differential control, by the total reference torque T ref The torque deviation ΔT obtained from the measured output total torque T is used as the input signal of PD control, and its output is the torque control value u, which is used for neural network learning deviation after preprocessing, and is used as the reference magnetic value after filtering. Part of the chain, the reference flux linkage output from the torque-flux linkage model is compensated.
[0062] Ⅱ. Dynamic RBF Neural Network Adaptive Inversion
[0063] The input signal of the RBF neural network in this example is the actual total flux linkage ψ(k-1) at the momen...
PUM
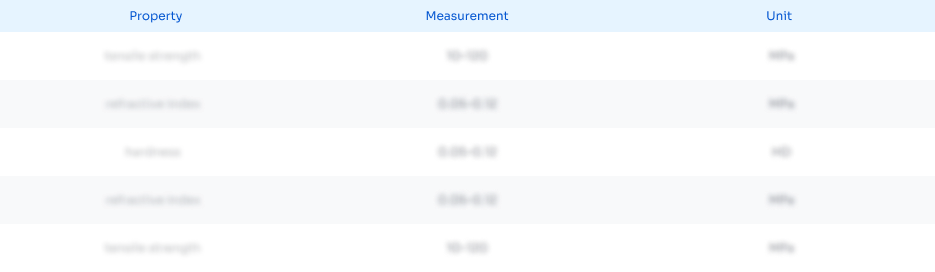
Abstract
Description
Claims
Application Information
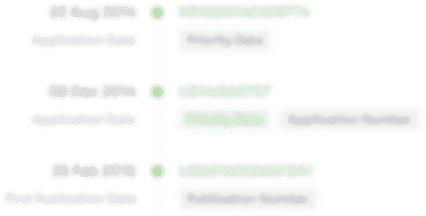
- R&D Engineer
- R&D Manager
- IP Professional
- Industry Leading Data Capabilities
- Powerful AI technology
- Patent DNA Extraction
Browse by: Latest US Patents, China's latest patents, Technical Efficacy Thesaurus, Application Domain, Technology Topic, Popular Technical Reports.
© 2024 PatSnap. All rights reserved.Legal|Privacy policy|Modern Slavery Act Transparency Statement|Sitemap|About US| Contact US: help@patsnap.com