A method for establishing a cldnn structure applied to end-to-end speech recognition
A technology for speech recognition and establishment methods, applied in speech recognition, speech analysis, neural learning methods, etc., which can solve problems such as gradient explosion, disappearance, and increasing gradients
- Summary
- Abstract
- Description
- Claims
- Application Information
AI Technical Summary
Problems solved by technology
Method used
Image
Examples
Embodiment Construction
[0049] The technical solutions in the embodiments of the present invention will be described clearly and in detail below with reference to the drawings in the embodiments of the present invention. The described embodiments are only some of the embodiments of the invention.
[0050] The technical scheme that the present invention solves the problems of the technologies described above is:
[0051] S1, dividing the speech data set, and dividing the data set into a training set, a cross-validation set and a test set;
[0052] S2, carry out preprocessing to all data, and then obtain the mel-frequency cepstral coefficient (MFCC) of speech signal, preprocessing step is:
[0053] Pre-emphasis: For the signal passing through the high-pass filter H(Z)=1-μz -1
[0054] Framing: divide the entire speech signal into small segments of 30ms per frame and 10ms frame shift.
[0055] Windowing: add a Hamming window to each frame signal
[0056] S'(n)=S(n)*W(n)
[0057] (a takes 0.46) ...
PUM
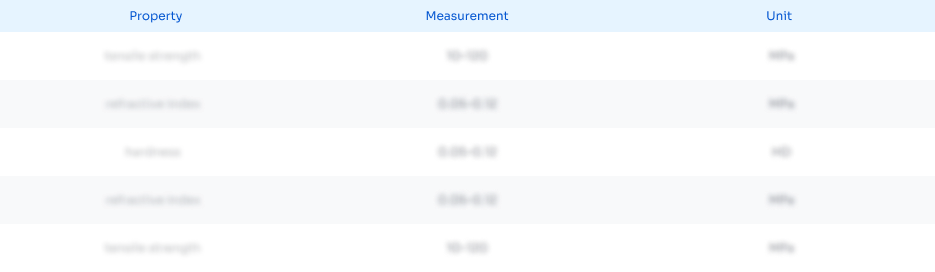
Abstract
Description
Claims
Application Information
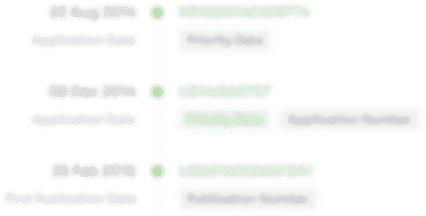
- R&D Engineer
- R&D Manager
- IP Professional
- Industry Leading Data Capabilities
- Powerful AI technology
- Patent DNA Extraction
Browse by: Latest US Patents, China's latest patents, Technical Efficacy Thesaurus, Application Domain, Technology Topic, Popular Technical Reports.
© 2024 PatSnap. All rights reserved.Legal|Privacy policy|Modern Slavery Act Transparency Statement|Sitemap|About US| Contact US: help@patsnap.com