Wheat grain resistant starch content rapid measurement method
A technology for resistant starch and wheat grain, applied in the direction of testing starch pollutants, testing food, measuring devices, etc., can solve problems such as time-consuming and complicated operations
- Summary
- Abstract
- Description
- Claims
- Application Information
AI Technical Summary
Problems solved by technology
Method used
Image
Examples
Embodiment Construction
[0029] The rapid determination method of the resistant starch content of the wheat grain comprises the following steps:
[0030] (1) Select a certain amount of grains of different wheat materials in the germplasm resource bank. Each material is clean wheat grains after manual removal of impurities, and grind the wheat grains into fine powder with a cyclone mill equipped with a 0.8 mm sieve plate , and then put the obtained wheat flour into a sealed bag, put it into a drying oven in an open shape and dry it at 40°C for 8 hours, take it out, cool it naturally and seal it for later use; in this way, whole wheat flour materials of different materials can be prepared .
[0031] (2) Using the K-RSTAR resistant starch kit produced by the Irish company, the resistant starch content of each whole wheat flour material was measured multiple times according to the AOAC method 2002.02 resistant starch determination principle, and then the average value of the multiple measurement results w...
PUM
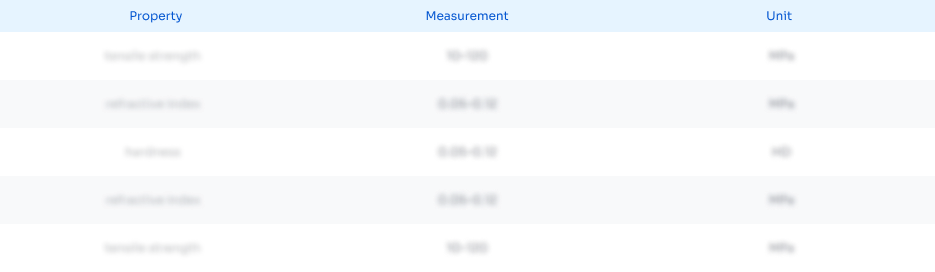
Abstract
Description
Claims
Application Information
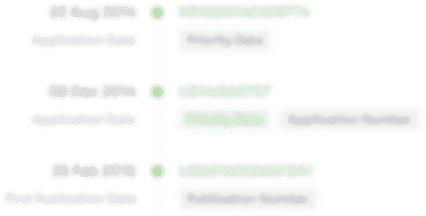
- R&D Engineer
- R&D Manager
- IP Professional
- Industry Leading Data Capabilities
- Powerful AI technology
- Patent DNA Extraction
Browse by: Latest US Patents, China's latest patents, Technical Efficacy Thesaurus, Application Domain, Technology Topic, Popular Technical Reports.
© 2024 PatSnap. All rights reserved.Legal|Privacy policy|Modern Slavery Act Transparency Statement|Sitemap|About US| Contact US: help@patsnap.com