An electromyographic signal classification method based on a two-parameter kernel optimization type extreme learning machine
An extreme learning machine and electromyographic signal technology, applied in the field of pattern recognition, can solve problems such as complex linear discrimination
- Summary
- Abstract
- Description
- Claims
- Application Information
AI Technical Summary
Problems solved by technology
Method used
Image
Examples
Embodiment Construction
[0036] like figure 1 As shown, this embodiment includes the following steps:
[0037] Step 1: Collect 4 channels of EMG signals and plantar pressure of the human gastrocnemius, tibialis anterior, vastus medialis, and vastus externus when the human body is doing daily actions;
[0038] Step 2, extract the VAR, WAMP, EWT, MA and plantar pressure AR coefficients of the 4 EMG signals;
[0039] Step 3, performing feature fusion on the 5 features of step 2 by combining the generalized canonical correlation method (WGA-GCCA) with genetic algorithm;
[0040] Step 4, determine the feedforward neural network structure of the extreme learning machine, and determine the number of neurons in the hidden layer;
[0041] Step five, randomly set the connection weight ω of the input layer and the hidden layer of the feedforward neural network and the bias b of the hidden layer neurons, and calculate the output matrix H of the hidden layer;
[0042] Step 6, find the least squares norm solutio...
PUM
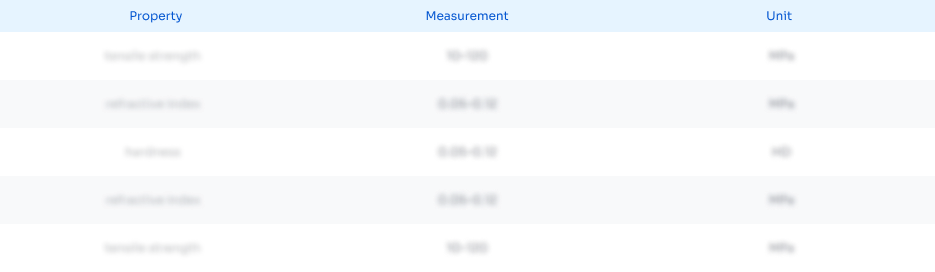
Abstract
Description
Claims
Application Information
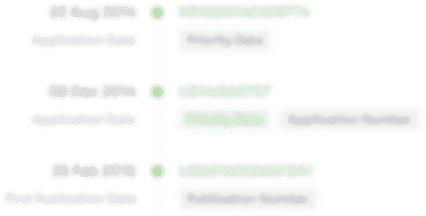
- R&D Engineer
- R&D Manager
- IP Professional
- Industry Leading Data Capabilities
- Powerful AI technology
- Patent DNA Extraction
Browse by: Latest US Patents, China's latest patents, Technical Efficacy Thesaurus, Application Domain, Technology Topic, Popular Technical Reports.
© 2024 PatSnap. All rights reserved.Legal|Privacy policy|Modern Slavery Act Transparency Statement|Sitemap|About US| Contact US: help@patsnap.com