Microbial marker of type II diabetes mellitus and application of microbial marker
A technology of microorganisms and markers, which is applied in the field of microorganisms, can solve the problems of being unable to display information related to type II diabetes, and achieve the effects of reducing the risk of type II diabetes, improving the intestinal environment, and being widely used
- Summary
- Abstract
- Description
- Claims
- Application Information
AI Technical Summary
Problems solved by technology
Method used
Image
Examples
Embodiment 1
[0043] The selection of embodiment 1 machine learning classifier
[0044] The present invention selects Xgboost as the basis of the optimal model through the screening and matching of a large amount of information, and the specific method is as follows:
[0045] (1) Perform 16S sequencing on the fecal bacteria of 66 cases of type 2 diabetes and 53 cases of healthy people, clean the data and match the database to obtain the abundance of 243 bacteria of specific genera, the distribution of experimental samples is shown in figure 2 ;
[0046] (2) Construct a machine learning classifier: the measured abundance values of 243 bacterial genera are used as input data, and the diagnosis results are used as target results (0: control, 1: suffering from type 2 diabetes disease)). The classifier constructed includes Logistic regression, random forest, Adaboost and Xgboost, and the classifier is adjusted with Gridsearch to select optimal parameters;
[0047] (3) Cross-validation selec...
Embodiment 2
[0049] The bacterium of embodiment 2 specific species is selected
[0050] (1) Feature screening: wrapping feature selection, that is, the evaluation principle of using the performance of the learner (Xgboost) that will be used eventually as a feature subset. The Xgboost model obtains the Feature-importance score of variable features, and the top 20 features and their importance scores are shown in Figure 4 , according to the high and low ranking of the score, gradually increase the number of bacterial variables to obtain the variables required for the optimal ROC-AUC (see Figure 5 );
[0051] (2) Test model: The bacterial abundance of 18 specific genera is used as the value of the input characteristic variable, and the diagnosis result is used as the target result. All data is cross-segmented by 5-fold stratified sampling, the training set is input into Xgboost, and the test set is used to test the prediction results of xgboost. The prediction results of each Xgboost are ...
PUM
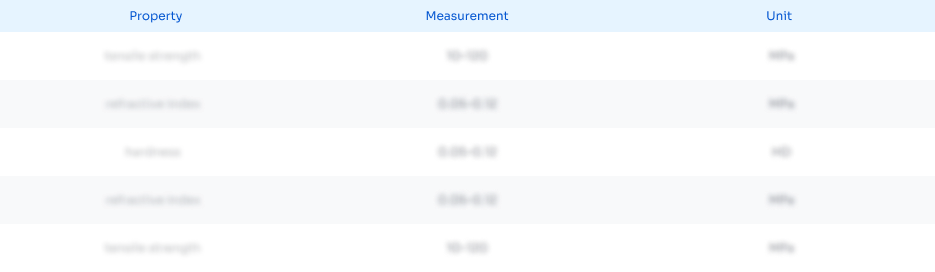
Abstract
Description
Claims
Application Information
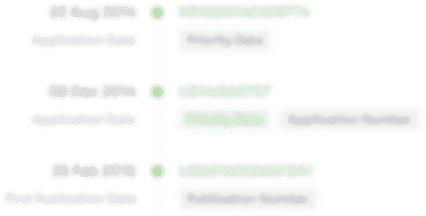
- R&D Engineer
- R&D Manager
- IP Professional
- Industry Leading Data Capabilities
- Powerful AI technology
- Patent DNA Extraction
Browse by: Latest US Patents, China's latest patents, Technical Efficacy Thesaurus, Application Domain, Technology Topic, Popular Technical Reports.
© 2024 PatSnap. All rights reserved.Legal|Privacy policy|Modern Slavery Act Transparency Statement|Sitemap|About US| Contact US: help@patsnap.com