Novel answer selection model based on GRU attention mechanism
A technology of attention and mechanism, applied in special data processing applications, instruments, unstructured text data retrieval, etc., can solve problems such as multi-noise, and achieve the effect of improving algorithm stability and algorithm stability
- Summary
- Abstract
- Description
- Claims
- Application Information
AI Technical Summary
Problems solved by technology
Method used
Image
Examples
Embodiment 1
[0027] figure 1 is a schematic structural diagram of a recurrent neural network RNN according to an embodiment of the present invention. The cyclic neural network can be expressed as a function, and the common form of the neural network can be divided into an input layer, a hidden layer, and an output layer. No matter how many layers there are in the hidden layer, it can be abstracted into a large hidden layer as a whole. The hidden layer can also be expressed in the form of a function, which takes the data of the input layer as an independent variable and calculates the output dependent variable. The output layer is also a function that takes the output of the dependent variable from the hidden layer as input. RNN has important applications in many natural language processing tasks. RNN has achieved very good results in tasks such as language model, text generation, machine translation, language recognition and image description generation.
[0028] according to figure ...
Embodiment
[0084] The present invention has carried out accuracy comparison and analysis experiment based on the attention mechanism model inside GRU to above-mentioned method, specifically as follows:
[0085] This experiment uses InsuranceQA dataset and WikiQA dataset.
[0086]Firstly, the InsuranceQA data set is used. The InsuranceQA data set is divided into three parts: training set, verification set and test set. The test set is divided into two small test sets (Test1 and Test2). Each part has the same compositional format: each question-answer pair consists of 1 question and 11 answers, where 11 answers include 1 correct answer and 10 distracting answers. In the model training phase, for each question in the training set, one of the corresponding 10 interference answers is randomly selected as the interference answer during training; in the testing phase, the distance between each question and its corresponding 11 answers is calculated. similarity score. Before the experiment, th...
PUM
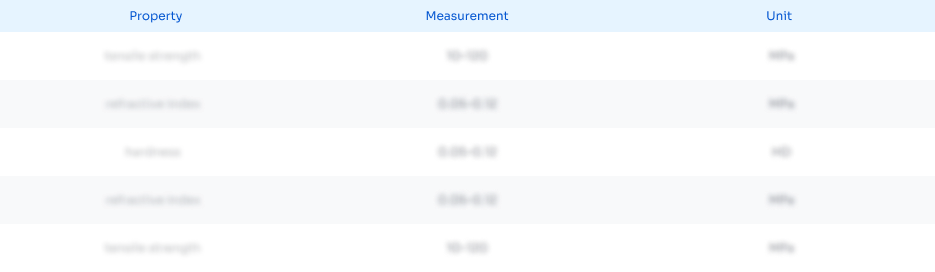
Abstract
Description
Claims
Application Information
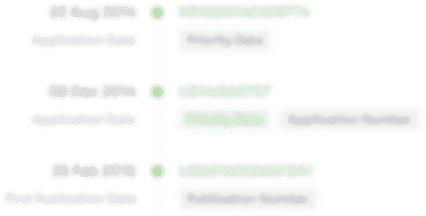
- R&D Engineer
- R&D Manager
- IP Professional
- Industry Leading Data Capabilities
- Powerful AI technology
- Patent DNA Extraction
Browse by: Latest US Patents, China's latest patents, Technical Efficacy Thesaurus, Application Domain, Technology Topic, Popular Technical Reports.
© 2024 PatSnap. All rights reserved.Legal|Privacy policy|Modern Slavery Act Transparency Statement|Sitemap|About US| Contact US: help@patsnap.com