Semantic Segmentation Method Based on Efficient Convolutional Networks and Convolutional Conditional Random Fields
A conditional random field and convolutional network technology, applied in biological neural network models, image analysis, image enhancement, etc., can solve problems such as expensive calculation costs and high accuracy, and achieve fine segmentation results, increased speed, and accurate segmentation results Effect
- Summary
- Abstract
- Description
- Claims
- Application Information
AI Technical Summary
Problems solved by technology
Method used
Image
Examples
Embodiment Construction
[0052] In order to more clearly illustrate the above-mentioned purpose, features and advantages of the present invention, the method network mentioned in the present invention will be described in more detail below in conjunction with the accompanying drawings and specific implementation methods.
[0053] The specific composition and steps of the neural network framework based on Efficient ConvNet and Convolutional CRFs proposed by the present invention are as follows (for ease of illustration, it is assumed that the input image size is 1024x512):
[0054] Step 1. Input an RGB image of any size, and use an encoder network composed of a downsampling module (Downsampler block) and a one-dimensional non-bottleneck unit (Non-bottleneck-1D) to extract the semantics of the original RGB image, and obtain an image consisting of A matrix of feature maps. The specific implementation is as follows:
[0055] Encode the input RGB image, the encoder such as image 3 In the "encoder" part,...
PUM
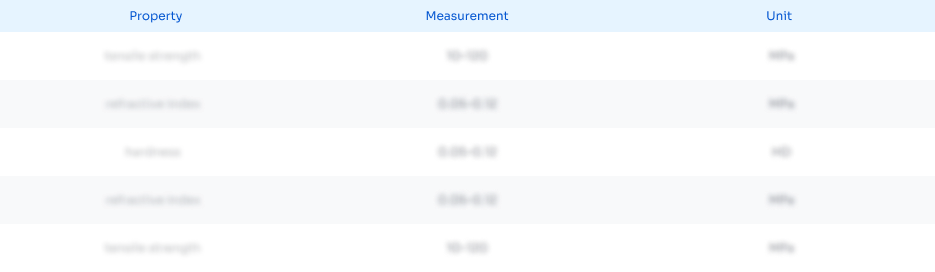
Abstract
Description
Claims
Application Information
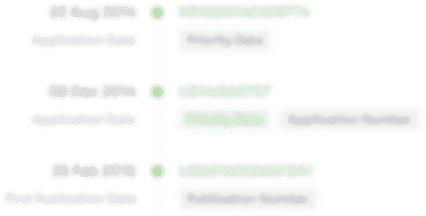
- R&D
- Intellectual Property
- Life Sciences
- Materials
- Tech Scout
- Unparalleled Data Quality
- Higher Quality Content
- 60% Fewer Hallucinations
Browse by: Latest US Patents, China's latest patents, Technical Efficacy Thesaurus, Application Domain, Technology Topic, Popular Technical Reports.
© 2025 PatSnap. All rights reserved.Legal|Privacy policy|Modern Slavery Act Transparency Statement|Sitemap|About US| Contact US: help@patsnap.com